Downlink channel estimation method for massive MIMO communication system based on real-valued sparse Bayesian learning
A sparse Bayesian, downlink technology, applied in the field of downlink channel estimation of massive MIMO communication systems, can solve problems such as excessive computational load, and achieve the effects of reducing computational load, saving computing time, and improving performance
- Summary
- Abstract
- Description
- Claims
- Application Information
AI Technical Summary
Problems solved by technology
Method used
Image
Examples
Embodiment Construction
[0021] The present invention will be further described below in conjunction with accompanying drawing.
[0022] Such as figure 1 Shown, concrete implementation steps and method of the present invention comprise as follows:
[0023] (1) The base station adopts a uniform linear array with N antennas, and the mobile user in the downlink uses a single antenna. In T time, the base station sends the pilot signal matrix X, and the signal received by the mobile user is y =Φ(β)s+n, where:
[0024] Φ(β)=XA(β) is called the measurement matrix,
[0025] A(β)=[a(θ 1 +β 1 ), a(θ 2 +β 2 ),...,a(θ N +β N )],
[0026]
[0027] φ(θ i +β i )=(2πd / λ)sin(θ i +β i ),
[0028] λ represents the operating wavelength of the electromagnetic wave, d represents the distance between adjacent sensors, Indicates an even division The L grid points of Element β in i represents theta i The angular deviation on
[0029] s is an L-dimensional channel sparsely represented vec...
PUM
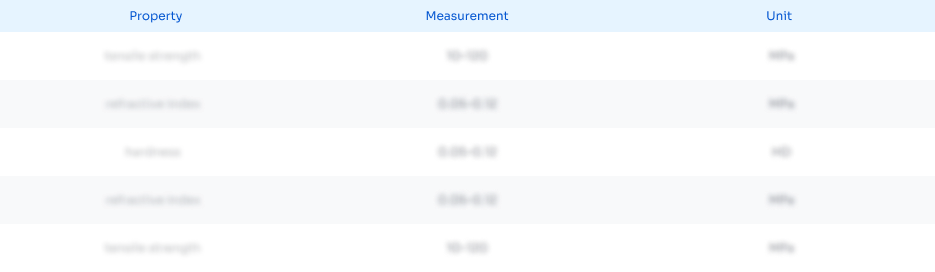
Abstract
Description
Claims
Application Information
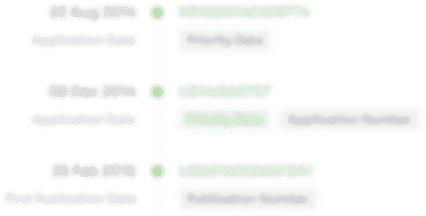
- Generate Ideas
- Intellectual Property
- Life Sciences
- Materials
- Tech Scout
- Unparalleled Data Quality
- Higher Quality Content
- 60% Fewer Hallucinations
Browse by: Latest US Patents, China's latest patents, Technical Efficacy Thesaurus, Application Domain, Technology Topic, Popular Technical Reports.
© 2025 PatSnap. All rights reserved.Legal|Privacy policy|Modern Slavery Act Transparency Statement|Sitemap|About US| Contact US: help@patsnap.com