Reinforcement learning unit matching recurrent neural network system and training and prediction method thereof
A recurrent neural network and reinforcement learning technology, applied in the field of reinforcement learning unit matching recurrent neural network system and its training and prediction, can solve the problem of unsatisfactory model prediction accuracy and computational efficiency, loss of sample validity and diversity, and inconsistent prediction results. Determine and other problems to avoid blind search, enhance generalization ability, and improve convergence speed
- Summary
- Abstract
- Description
- Claims
- Application Information
AI Technical Summary
Problems solved by technology
Method used
Image
Examples
Embodiment Construction
[0037] A reinforcement learning unit matching cycle neural network system, including a cycle neural network and a reinforcement learning unit, the cycle neural network includes input, output and a plurality of hidden layers, the hidden layer includes a plurality of hidden layer nodes, and also includes a monotonic trend Recognizer, the monotone trend recognizer judges the trend state of the time series constructed by the input, and the reinforcement learning unit is based on the trend state of the input time series, and selects a cycle in which the number of hidden layers and the number of nodes in the hidden layer are adapted to its changing law A neural network, wherein the trend state of the input time series includes multiple states, and each trend state corresponds to a cyclic neural network in which the number of hidden layers and the number of nodes in the hidden layer are adapted to its changing law.
[0038] In this embodiment, the trend state includes an upward trend ...
PUM
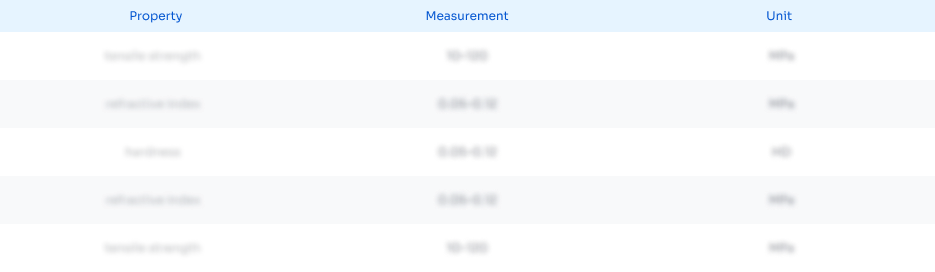
Abstract
Description
Claims
Application Information
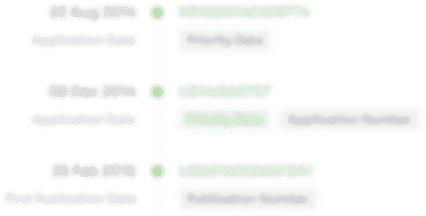
- R&D Engineer
- R&D Manager
- IP Professional
- Industry Leading Data Capabilities
- Powerful AI technology
- Patent DNA Extraction
Browse by: Latest US Patents, China's latest patents, Technical Efficacy Thesaurus, Application Domain, Technology Topic, Popular Technical Reports.
© 2024 PatSnap. All rights reserved.Legal|Privacy policy|Modern Slavery Act Transparency Statement|Sitemap|About US| Contact US: help@patsnap.com