Multi-target detection method based on a cascade hourglass neural network
A neural network and detection method technology, applied in the field of multi-target detection, can solve problems such as poor effect and slow speed, and achieve the effect of improving detection accuracy and fast operation speed
- Summary
- Abstract
- Description
- Claims
- Application Information
AI Technical Summary
Problems solved by technology
Method used
Image
Examples
Embodiment Construction
[0021] The specific implementation manners of the present invention will be described in detail below in conjunction with the accompanying drawings. However, the following examples are only used to illustrate the present invention in detail, and do not limit the scope of the present invention in any way.
[0022] Such as Figure 4 As shown, a kind of multi-target detection method based on cascaded hourglass neural network of the present invention comprises the following steps:
[0023] Step 1, such as figure 1 As shown, collect training samples: use the image acquisition equipment to detect the target image, mark the target image and do preprocessing to make the image meet the format requirements, and build a training sample set; image preprocessing uses image enhancement, including random angle ( -180°, 180°) rotation and random size scaling (0.5x-2x).
[0024] Step 2. Build a deep learning framework and build a backbone network cascaded hourglass network for target detect...
PUM
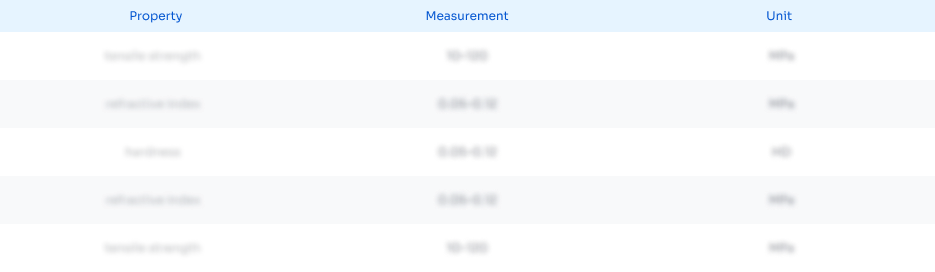
Abstract
Description
Claims
Application Information
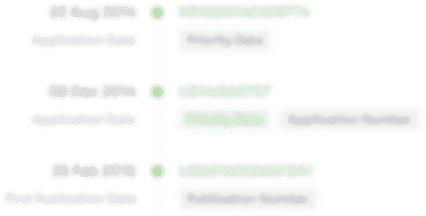
- Generate Ideas
- Intellectual Property
- Life Sciences
- Materials
- Tech Scout
- Unparalleled Data Quality
- Higher Quality Content
- 60% Fewer Hallucinations
Browse by: Latest US Patents, China's latest patents, Technical Efficacy Thesaurus, Application Domain, Technology Topic, Popular Technical Reports.
© 2025 PatSnap. All rights reserved.Legal|Privacy policy|Modern Slavery Act Transparency Statement|Sitemap|About US| Contact US: help@patsnap.com