Oil-water relative permeability curve calculation method based on machine learning
A relative permeability and calculation method technology, applied in the field of oilfield development data mining, can solve problems such as large amount of data, inability to reflect relevant factors, inability to fully reflect the actual situation, etc., to achieve the effect of ensuring accuracy and simple and efficient solution method
- Summary
- Abstract
- Description
- Claims
- Application Information
AI Technical Summary
Problems solved by technology
Method used
Image
Examples
Embodiment 1
[0097] Select a group of samples in the data set and describe some parameters: the sedimentary microfacies are channel microfacies; the main mineral components are feldspar, quartz and muscovite; the permeability is 380mDc, the porosity is 0.34; the viscosity of oil and water is 0.52x10 -3 / Pa.s, 1280.6x10 -3 / Pa.s and other parameters.
[0098] After steps S1 and S2, the sample is standardized, and the above neural Turing machine is called to quickly obtain the output of the characteristic value of the oil-water relative permeability curve; according to the output result, the data is restored to draw the oil-water relative permeability curve, as shown in Figure 11 As shown, the prediction effect is ideal, and the oil-water relative permeability curve can well reflect the situation of the entire reservoir.
PUM
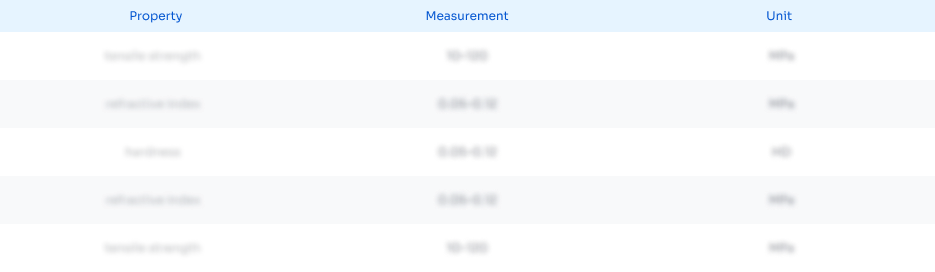
Abstract
Description
Claims
Application Information
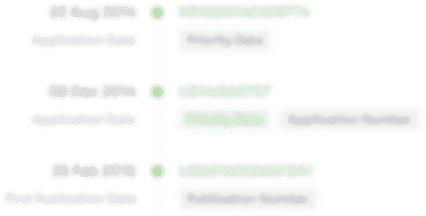
- R&D Engineer
- R&D Manager
- IP Professional
- Industry Leading Data Capabilities
- Powerful AI technology
- Patent DNA Extraction
Browse by: Latest US Patents, China's latest patents, Technical Efficacy Thesaurus, Application Domain, Technology Topic, Popular Technical Reports.
© 2024 PatSnap. All rights reserved.Legal|Privacy policy|Modern Slavery Act Transparency Statement|Sitemap|About US| Contact US: help@patsnap.com