A Faster RCNN Object Detection Method Based on Difficult Sample Mining
A target detection and sample technology, applied in instruments, computing, character and pattern recognition, etc., can solve the problems of poor sample training effect, low feature matching accuracy, long detection time, etc., to increase sample diversity, improve search ability, The effect of improving generalization ability
- Summary
- Abstract
- Description
- Claims
- Application Information
AI Technical Summary
Problems solved by technology
Method used
Image
Examples
Embodiment Construction
[0056] Aiming at the sample problem, the present invention provides a combination of online sample mining technology and negative hard-to-segment sample mining without adding samples, so that the model can learn its characteristics in a targeted manner for existing hard-to-segment samples, To achieve the effect of further improving the generalization and robustness of the model.
[0057] To achieve the above object, the present invention adopts the following technical solutions:
[0058] A Faster RCNN object detection method based on difficult sample mining, comprising the following steps:
[0059] Step 1, image target detection based on deep learning;
[0060] At present, most image target detection models based on deep learning are based on convolutional neural networks, so the present invention mainly analyzes based on Faster RCNN, and proposes a reasonable improved method.
[0061] Faster RCNN uses Softmax Loss and Smooth L1 Loss to jointly train classification probabili...
PUM
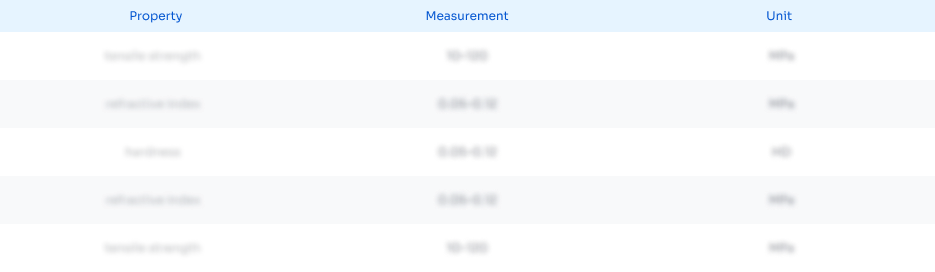
Abstract
Description
Claims
Application Information
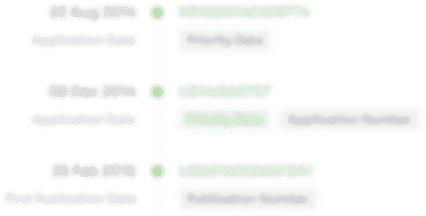
- R&D
- Intellectual Property
- Life Sciences
- Materials
- Tech Scout
- Unparalleled Data Quality
- Higher Quality Content
- 60% Fewer Hallucinations
Browse by: Latest US Patents, China's latest patents, Technical Efficacy Thesaurus, Application Domain, Technology Topic, Popular Technical Reports.
© 2025 PatSnap. All rights reserved.Legal|Privacy policy|Modern Slavery Act Transparency Statement|Sitemap|About US| Contact US: help@patsnap.com