Fault diagnosis method based on compressed sensing and improved multi-scale network
A fault diagnosis and compressive sensing technology, applied in biological neural network models, testing of mechanical components, testing of machine/structural components, etc., can solve problems such as difficulties in the optimization process of multi-scale network diagnosis, difficulty in applying small samples, etc. The effect of optimizing the process is difficult, improving the accuracy, and improving the efficiency of data analysis
- Summary
- Abstract
- Description
- Claims
- Application Information
AI Technical Summary
Problems solved by technology
Method used
Image
Examples
Embodiment 1
[0066] Example 1: Validity Verification
[0067] In order to verify the effectiveness of the fault diagnosis method proposed in the present invention, this embodiment uses the public experimental data set provided by the Bearing Data Center of Case Western Reserve University in the United States for verification. The experimental bench includes: a driving motor, bearing at the driving end, a switch encoder, and a dynamometer. Set the speed of the driving motor at 1750 rpm and the load at 2Hp. Collect the vibration signal at the 6 o'clock position at the driving motor end. The sampling rate is 12kHz. The fault types of rolling bearings are inner ring defects, outer ring defects and ball defects. The severity of the faults is simulated by EDM, and the fault diameters are 7, 14, and 21 mils (mil) respectively. The details of the 10 bearing condition types are shown in Table 1. 2400 data samples are collected under each bearing state type, and each data sample contains 4096 data...
Embodiment 2
[0083] Example 2: Hyperparameter Settings
[0084] The present invention uses compressed sensing technology to compress raw sensor data, which improves data efficiency to a certain extent, and studies the performance of data-driven fault diagnosis methods using different hyperparameters in the case of compressed sensing, including calculation time and classification Accuracy; Comparing computation time with and without Compressed Sensing. Its specific implementation steps are as follows figure 1 As shown, the parameter settings of the deep learning process are shown in Table 3. A total of 10 repeated experiments were carried out, and the average results were calculated and used for the data.
[0085] table 3
[0086]
[0087] Experimental results such as Image 6 , Figure 7 As shown, the experimental results show that for a specific number of feature maps C, as the number of neurons in the output layer increases, the classification accuracy of rolling bearings increase...
Embodiment 3
[0094] Example 3: Small sample problem
[0095] Generally, the performance of deep model-based data-driven fault diagnosis methods depends on the size of the sensing dataset, and when more training datasets are used, the accuracy of fault diagnosis increases, however, it is difficult to obtain sufficient monitoring sensor data. The present invention first uses the measurement matrix of compressed sensing to generate enough training samples for subsequent deep learning analysis. Its specific implementation steps are as follows figure 1 As shown, the parameter settings of the small sample training process are shown in Table 6. A total of 10 repeated experiments were carried out, and the average results were calculated and used for the data.
[0096] Different bearing state types (see Table 1) randomly select 200 training samples, so the total number of training samples for 10 bearing state types is 2000. In this example, the Gaussian measurement matrix is used to generate e...
PUM
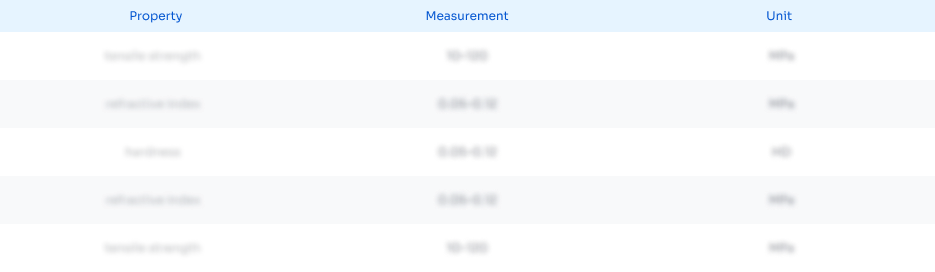
Abstract
Description
Claims
Application Information
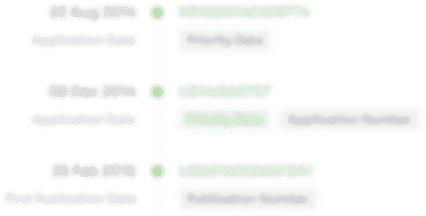
- R&D
- Intellectual Property
- Life Sciences
- Materials
- Tech Scout
- Unparalleled Data Quality
- Higher Quality Content
- 60% Fewer Hallucinations
Browse by: Latest US Patents, China's latest patents, Technical Efficacy Thesaurus, Application Domain, Technology Topic, Popular Technical Reports.
© 2025 PatSnap. All rights reserved.Legal|Privacy policy|Modern Slavery Act Transparency Statement|Sitemap|About US| Contact US: help@patsnap.com