Driver fatigue detection based on the long-term and short-term memory network
A driver fatigue, long-term and short-term memory technology, applied in the field of deep learning and image processing, can solve problems such as difficult large-scale promotion, poor operability, fatigue characteristics and fatigue degree correlation are not easy to be quantified, etc., to achieve robustness Excellent performance and accuracy, avoiding the image processing process, and high fatigue detection accuracy
- Summary
- Abstract
- Description
- Claims
- Application Information
AI Technical Summary
Problems solved by technology
Method used
Image
Examples
Embodiment Construction
[0022] The present invention will be further described in detail below in combination with specific embodiments.
[0023] The overall framework schematic diagram of the present invention is as figure 1 As shown, firstly, the infrared video acquisition system is used to collect the face video images of the driver in the three situations of not wearing glasses, wearing sunglasses, and wearing myopia glasses; Feature point location, according to the geometric relationship between the feature points to obtain the driver's eye image sequence; next, an end-to-end convolutional recurrent neural network model is designed to extract the spatial and temporal features of the driver's eye state over a period of time , and finally make a fatigue judgment based on the contextual relationship between the features of the adjacent image frames of the eye.
[0024] The specific implementation process of the technical solution of the present invention will be described below in conjunction with...
PUM
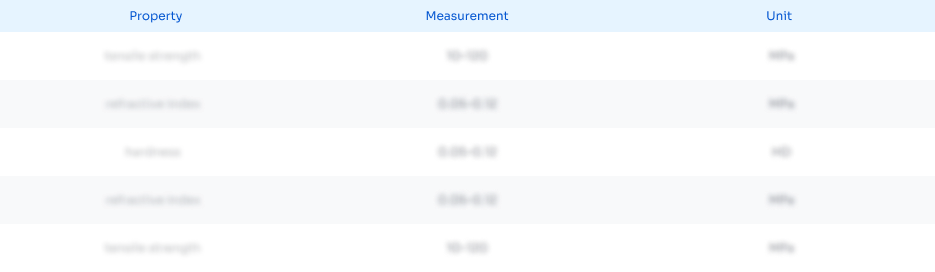
Abstract
Description
Claims
Application Information
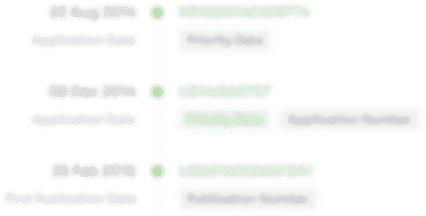
- R&D Engineer
- R&D Manager
- IP Professional
- Industry Leading Data Capabilities
- Powerful AI technology
- Patent DNA Extraction
Browse by: Latest US Patents, China's latest patents, Technical Efficacy Thesaurus, Application Domain, Technology Topic, Popular Technical Reports.
© 2024 PatSnap. All rights reserved.Legal|Privacy policy|Modern Slavery Act Transparency Statement|Sitemap|About US| Contact US: help@patsnap.com