A lightweight reconstruction method for missing spatio-temporal data
A technology for spatiotemporal data and missing data, applied in neural learning methods, neural architectures, complex mathematical operations, etc., can solve problems such as slow local optimal solutions, missing spatiotemporal data reconstruction accuracy and computational efficiency, and training speed without consideration
- Summary
- Abstract
- Description
- Claims
- Application Information
AI Technical Summary
Problems solved by technology
Method used
Image
Examples
Embodiment Construction
[0050] The present invention will be further described in detail below in conjunction with the accompanying drawings and specific embodiments.
[0051] A lightweight reconstruction method for missing spatio-temporal data, including the following steps:
[0052] Step 1. Spatio-temporal data representation;
[0053] Through continuous sampling of spatial objects in fixed spatial positions, static reference point data and network data are generated, such as environmental pollution data monitored by fixed sensors, and historical traffic condition data generated by floating vehicles driving on the road network. The sampling process of these two types of data is spatially synchronized and preprocessed at the same time interval for subsequent modeling. They have common characteristics, that is, space static and time dynamic, so they are abstracted into a unified space-time state matrix to represent. Assuming that the number of sampled spatial objects is M, and the length of the his...
PUM
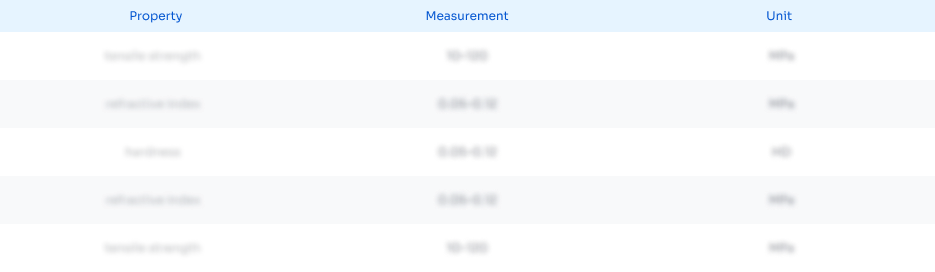
Abstract
Description
Claims
Application Information
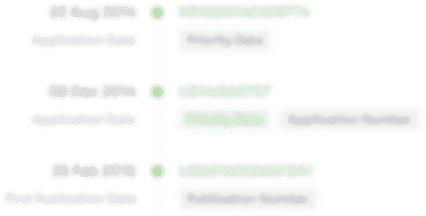
- R&D Engineer
- R&D Manager
- IP Professional
- Industry Leading Data Capabilities
- Powerful AI technology
- Patent DNA Extraction
Browse by: Latest US Patents, China's latest patents, Technical Efficacy Thesaurus, Application Domain, Technology Topic, Popular Technical Reports.
© 2024 PatSnap. All rights reserved.Legal|Privacy policy|Modern Slavery Act Transparency Statement|Sitemap|About US| Contact US: help@patsnap.com