A salient object detection method based on deep network layering and multi-task training
An object detection and deep network technology, applied in the field of image processing and computer vision, can solve the problem of insufficient details of the edge of the object
- Summary
- Abstract
- Description
- Claims
- Application Information
AI Technical Summary
Problems solved by technology
Method used
Image
Examples
Embodiment 1
[0033] In the first step, one or more tasks associated with the salient object detection task prepare training pictures required for multi-task training. Among them, for the saliency detection task, the training picture includes the original image and its corresponding saliency map, and for other tasks, the training picture includes the original image and its corresponding real result. Other tasks refer to one or more tasks that are intrinsically related to the saliency detection task and can share features, such as semantic segmentation, human gaze point prediction, etc. For semantic segmentation tasks, the training image includes the original image and the class label map to which the region in the image belongs.
[0034] The second step is to design a deep neural network architecture and loss function with hierarchical features. Specific steps include:
[0035] S2-1: Design the network structure, which includes a main forward network and multiple side paths connected to s...
Embodiment 2
[0041] The difference between embodiment 2 and embodiment 1 is that the task associated with the salient object detection task is the human gaze point prediction task, and the multi-task training pictures adopted in the joint training include the original image and the human eye gaze point prediction task picture, and the hierarchical The loss function corresponding to the human gaze point prediction task in the feature deep neural network architecture is the cross-entropy loss function, and the corresponding hierarchical feature deep neural network architecture is as follows: Figure 4 shown.
[0042] Other methods and steps are the same as in Embodiment 1, and will not be repeated here.
[0043] Based on the deep neural network architecture with hierarchical features in Embodiment 1, other tasks are expanded in the manner of this embodiment, and the formed network architecture should fall within the scope of protection of the present invention.
Embodiment 3
[0045] The difference between embodiment 2 and embodiment 1 is that the task associated with the salient object detection task uses multiple tasks, the multiple tasks are human gaze point prediction tasks and semantic segmentation tasks, and the corresponding deep neural network architecture of hierarchical features Such as Figure 5 shown.
[0046] The first step is to prepare the training pictures required for multi-task training. Among them, for the saliency detection task, the training picture includes the original image and its corresponding saliency map; for the semantic segmentation task, the training picture includes the original image and the class label map to which the area in the picture belongs; for the human gaze point prediction task, the training The picture contains the original picture and the prediction picture of human gaze point.
[0047] The second step is to design a deep neural network architecture and loss function with hierarchical features. Specif...
PUM
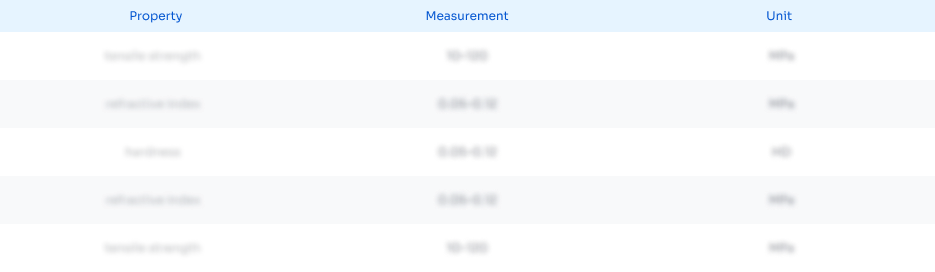
Abstract
Description
Claims
Application Information
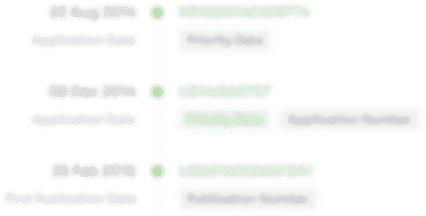
- Generate Ideas
- Intellectual Property
- Life Sciences
- Materials
- Tech Scout
- Unparalleled Data Quality
- Higher Quality Content
- 60% Fewer Hallucinations
Browse by: Latest US Patents, China's latest patents, Technical Efficacy Thesaurus, Application Domain, Technology Topic, Popular Technical Reports.
© 2025 PatSnap. All rights reserved.Legal|Privacy policy|Modern Slavery Act Transparency Statement|Sitemap|About US| Contact US: help@patsnap.com