A region-of-interest compressed sensing image reconstruction method based on deep learning
A region of interest, compressed sensing technology, applied in the field of compressed sensing image reconstruction of the region of interest, it can solve the problems of affecting the speed of the algorithm, high time complexity, unable to color image compressed sensing, etc., and achieve the effect of high reconstruction speed.
- Summary
- Abstract
- Description
- Claims
- Application Information
AI Technical Summary
Problems solved by technology
Method used
Image
Examples
Embodiment Construction
[0041] The present invention will be further described below in conjunction with the accompanying drawings.
[0042] refer to figure 1 , to further describe the specific implementation steps of the present invention.
[0043] Step 1. Construct the region-of-interest-aware reconstruction network.
[0044] The region-of-interest extraction sub-network in the region-of-interest-aware reconstruction network is constructed, which includes an eight-layer initial unified observation recovery module and a six-layer salient target region extraction module.
[0045] The structure of the initial unified observation recovery module is as follows: first convolution layer→deconvolution layer→second convolution layer→first residual block→second residual block→third residual Block → fifth convolutional layer → sixth convolutional layer.
[0046] Set the parameters of each layer of the initial unified observation recovery module.
[0047]The parameters of each layer of the first unified ob...
PUM
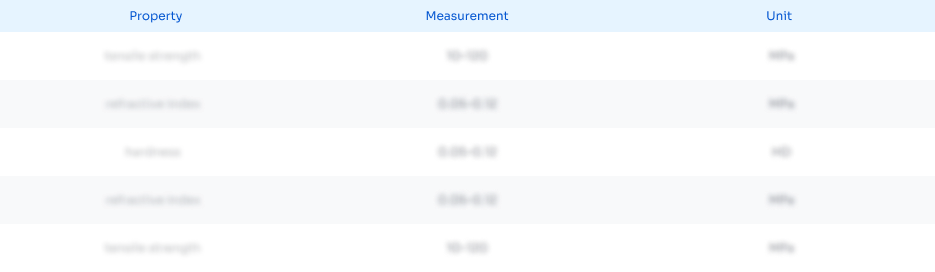
Abstract
Description
Claims
Application Information
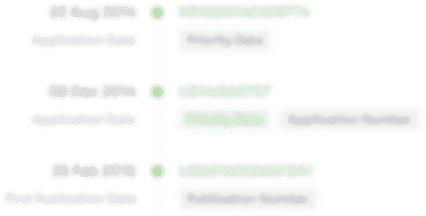
- R&D Engineer
- R&D Manager
- IP Professional
- Industry Leading Data Capabilities
- Powerful AI technology
- Patent DNA Extraction
Browse by: Latest US Patents, China's latest patents, Technical Efficacy Thesaurus, Application Domain, Technology Topic.
© 2024 PatSnap. All rights reserved.Legal|Privacy policy|Modern Slavery Act Transparency Statement|Sitemap