Data center resource offline scheduling method based on deep reinforcement learning
A reinforcement learning, data center technology, applied in the computer field to achieve good applicability
- Summary
- Abstract
- Description
- Claims
- Application Information
AI Technical Summary
Problems solved by technology
Method used
Image
Examples
Embodiment Construction
[0014] The specific implementation manners of the present invention will be described in further detail below according to the drawings and examples. The following examples are used to illustrate the present invention, but are not intended to limit the scope of the present invention.
[0015] figure 1 It is a schematic diagram of the deep reinforcement learning framework of the embodiment of the present invention.
[0016] Such as figure 1 As shown, the agent is interacting with the environment. At each time step t, the agent observes some state s_t and chooses an action a_t. After the action, the environment state transitions to s_(t+1), and the agent receives reward r_t. State transitions and rewards are stochastic and assumed to have Markov properties.
[0017] Further, the agent can only control its own behavior and has no prior knowledge of which state the environment will transition to or what the reward might be. During training, the agent can observe these quanti...
PUM
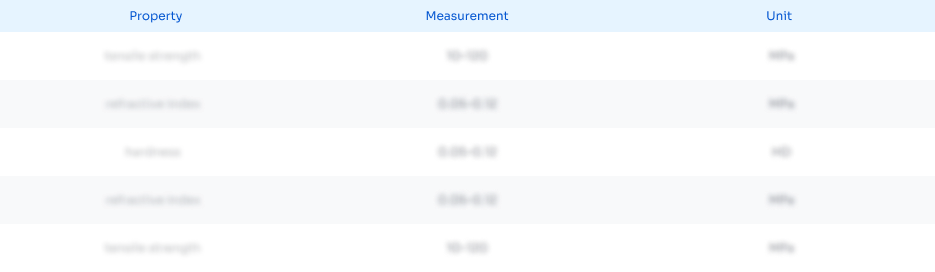
Abstract
Description
Claims
Application Information
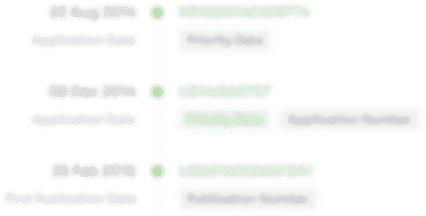
- R&D Engineer
- R&D Manager
- IP Professional
- Industry Leading Data Capabilities
- Powerful AI technology
- Patent DNA Extraction
Browse by: Latest US Patents, China's latest patents, Technical Efficacy Thesaurus, Application Domain, Technology Topic, Popular Technical Reports.
© 2024 PatSnap. All rights reserved.Legal|Privacy policy|Modern Slavery Act Transparency Statement|Sitemap|About US| Contact US: help@patsnap.com