Big data sensitive characteristic optimization selecting based equipment failure early warning method and system
A technology for sensitive features and equipment failures, applied in measuring devices, instruments, measuring ultrasonic/sonic/infrasonic waves, etc., and can solve problems such as low efficiency and low accuracy
- Summary
- Abstract
- Description
- Claims
- Application Information
AI Technical Summary
Problems solved by technology
Method used
Image
Examples
Embodiment 1
[0066] Such as figure 1 As shown, a device failure early warning method based on the optimal selection of sensitive features of big data, the steps are as follows:
[0067] Step 1: Collect vibration data, process data and electrical data under normal working conditions of the equipment.
[0068] Vibration data refers to the data contained in the vibration signal generated when the device is working. Process data such as temperature, pressure and flow data; electrical data such as current and voltage data.
[0069] Step 2: Select the vibration data in Step 1, and perform feature extraction on it to obtain 15 feature quantities.
[0070] The 15 feature quantities obtained from the feature extraction of the vibration data in the step 2 and step 4 include peak value, peak-to-peak value, average amplitude, square root amplitude, effective value, 1-octave amplitude, and 2-octave amplitude , 3-octave amplitude, waveform index, pulse index, peak index, margin index, skewness index,...
Embodiment 2
[0125] Such as image 3 As shown, an equipment failure early warning system based on the optimal selection of sensitive features of big data, including: data acquisition module, feature quantity extraction module, early warning model training module and early warning module, data collection module and feature quantity extraction module and early warning model training module respectively The modules are connected, the feature quantity extraction module is connected with the early warning model training module, and the early warning model training module is connected with the early warning module.
[0126] The data acquisition module is used to collect vibration parameters, process parameters and electrical parameters of the equipment unit;
[0127] The feature quantity extraction module is used for feature extraction of vibration parameters under normal working conditions and test working conditions of the equipment;
[0128] The early warning model training module is used to...
PUM
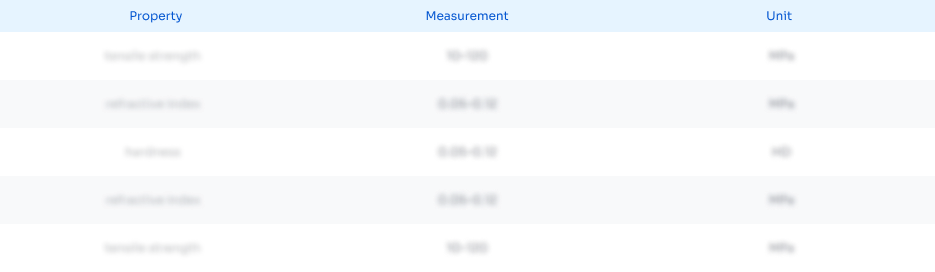
Abstract
Description
Claims
Application Information
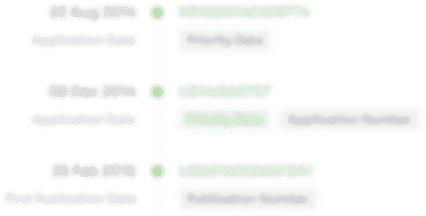
- R&D Engineer
- R&D Manager
- IP Professional
- Industry Leading Data Capabilities
- Powerful AI technology
- Patent DNA Extraction
Browse by: Latest US Patents, China's latest patents, Technical Efficacy Thesaurus, Application Domain, Technology Topic.
© 2024 PatSnap. All rights reserved.Legal|Privacy policy|Modern Slavery Act Transparency Statement|Sitemap