Cloud workflow task execution time prediction method based on limit gradient improvement
A technology for task execution and time prediction, applied in the field of cloud computing, can solve the problems of missing value variability, dependence on observations, poor robustness, etc., and achieve the effect of long training time
- Summary
- Abstract
- Description
- Claims
- Application Information
AI Technical Summary
Problems solved by technology
Method used
Image
Examples
Embodiment
[0091] The experiment collects 5112 groups of cloud workflow task execution time and all corresponding influencing factors data. Firstly, 4090 sets of data are extracted from it to form a training set, and the remaining 1022 sets of data are used as a test set, and the random forest model is used to process the data missing value of the training set and the test set respectively; secondly, based on the training set with complete data values, the extreme gradient is used. The algorithm trains the cloud workflow task execution time prediction model; finally, input the actual influencing factor data in the test set into the trained model, predict the corresponding cloud workflow task execution time, and compare it with the actual task execution time to calculate the prediction error.
[0092] The performance evaluation of the cloud workflow task execution time prediction model uses the root mean square error RMSE (root mean square error) as an indicator to evaluate the accuracy o...
PUM
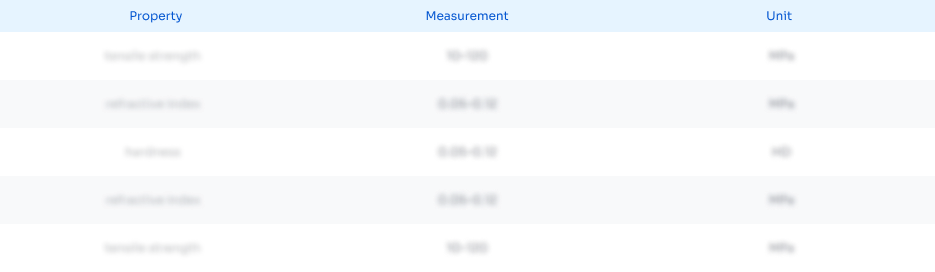
Abstract
Description
Claims
Application Information
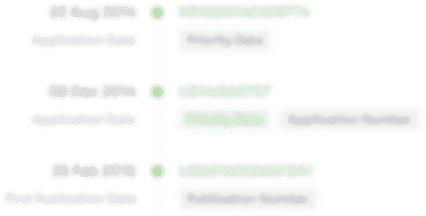
- R&D Engineer
- R&D Manager
- IP Professional
- Industry Leading Data Capabilities
- Powerful AI technology
- Patent DNA Extraction
Browse by: Latest US Patents, China's latest patents, Technical Efficacy Thesaurus, Application Domain, Technology Topic, Popular Technical Reports.
© 2024 PatSnap. All rights reserved.Legal|Privacy policy|Modern Slavery Act Transparency Statement|Sitemap|About US| Contact US: help@patsnap.com