Multi-source remote sensing image classification method based on two-way attention fusion neural network
A remote sensing image and classification method technology, applied in the field of image processing, can solve the problems of inability to communicate and fuse feature information, feature redundancy, and high feature dimension, and achieve accurate fusion classification results, reduce redundancy and dimension, and provide rich information Effect
- Summary
- Abstract
- Description
- Claims
- Application Information
AI Technical Summary
Problems solved by technology
Method used
Image
Examples
Embodiment Construction
[0029] The specific examples and effects of the present invention will be described in further detail below in conjunction with the accompanying drawings.
[0030] refer to figure 1 , the implementation steps of this example are as follows:
[0031] Step 1. Input a registered hyperspectral image and a LiDAR image to construct a training sample set and a test sample set.
[0032] 1a) Input a hyperspectral remote sensing image, which contains M marked pixels and N unmarked pixels, each pixel of the image is a sample, and M marked samples form a hyperspectral training sample set H, N Unmarked samples constitute the hyperspectral test sample set He. In this example, the number M of marked samples is 66485, and the number N of unmarked samples is 598360;
[0033] 1b) Input the lidar LiDAR image, where the number of marked pixels is M, and the coordinates are the same as those of the marked pixels in the hyperspectral image, the number of unmarked pixels is N, and the coordinates ...
PUM
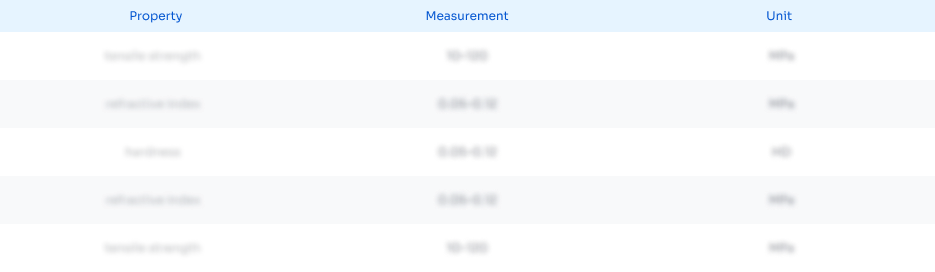
Abstract
Description
Claims
Application Information
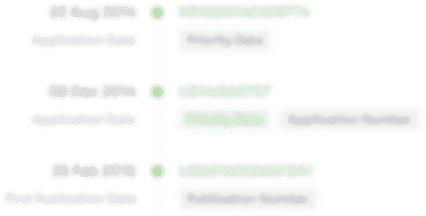
- R&D Engineer
- R&D Manager
- IP Professional
- Industry Leading Data Capabilities
- Powerful AI technology
- Patent DNA Extraction
Browse by: Latest US Patents, China's latest patents, Technical Efficacy Thesaurus, Application Domain, Technology Topic, Popular Technical Reports.
© 2024 PatSnap. All rights reserved.Legal|Privacy policy|Modern Slavery Act Transparency Statement|Sitemap|About US| Contact US: help@patsnap.com