Attention mechanism relationship comparison network model method based on small sample learning
A small sample, attention technology, applied in neural learning methods, biological neural network models, computer components, etc., can solve problems such as difficult model classification accuracy
- Summary
- Abstract
- Description
- Claims
- Application Information
AI Technical Summary
Problems solved by technology
Method used
Image
Examples
Embodiment Construction
[0082] Below in conjunction with accompanying drawing, further describe the present invention through embodiment, but do not limit the scope of the present invention in any way.
[0083] This paper proposes an attention mechanism relational contrastive network learning method based on small sample learning—ARCN method. The network realizes end-to-end learning. By introducing spectral normalization and attention mechanism in the convolutional neural network, the feature information of the small sample image is extracted, and the feature information is further recombined. Finally, the relationship between the features is realized through the relationship encoding module. The learning of inter-depth metric methods compares the relationship between images to achieve higher accuracy and more stable training for small sample images. image 3 It is the overall flowchart of the realization of the present invention.
[0084] The following embodiments are aimed at the small-sample publ...
PUM
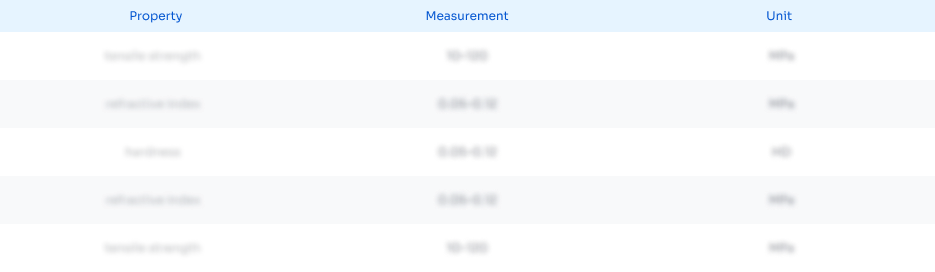
Abstract
Description
Claims
Application Information
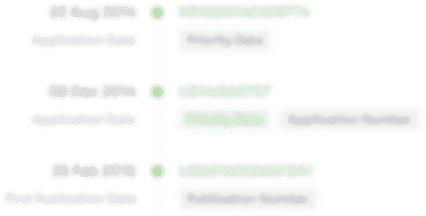
- R&D Engineer
- R&D Manager
- IP Professional
- Industry Leading Data Capabilities
- Powerful AI technology
- Patent DNA Extraction
Browse by: Latest US Patents, China's latest patents, Technical Efficacy Thesaurus, Application Domain, Technology Topic, Popular Technical Reports.
© 2024 PatSnap. All rights reserved.Legal|Privacy policy|Modern Slavery Act Transparency Statement|Sitemap|About US| Contact US: help@patsnap.com