Depth video behavior identification method and system
A technology of deep video and recognition methods, applied in character and pattern recognition, instruments, computing, etc., can solve the problems of ignoring learning ability, reducing the strong expressive ability of CNNs convolutional features, etc., to achieve the effect of good geometric information and privacy
- Summary
- Abstract
- Description
- Claims
- Application Information
AI Technical Summary
Problems solved by technology
Method used
Image
Examples
Embodiment 1
[0061] In one or more embodiments, a deep video behavior recognition method that fuses convolutional neural networks and channel and spatiotemporal interest point attention models is disclosed, such as figure 1 As shown, the dynamic image sequence representation of the depth video is used as the input of CNNs, and the channel and spatiotemporal interest point attention model are embedded after the CNNs convolutional layer, and the convolutional feature map is optimized and adjusted. Finally, the global average pooling is applied to the adjusted convolutional feature map of the input depth video to generate a feature representation of the behavioral video, which is input into the LSTM network to capture the temporal information of human behavior and classify it.
[0062] This embodiment proposes a dynamic image sequence representation (dynamic image sequence, DIS) for the video, divides the entire video into a group of short-term segments along the time axis, and then encodes ea...
Embodiment 2
[0160] In one or more embodiments, a deep video behavior recognition system that fuses convolutional neural networks and channel and spatiotemporal interest point attention models is disclosed, including a server, which includes a memory, a processor, and a memory stored on the memory And it is a computer program that can run on a processor, and when the processor executes the program, the depth video behavior recognition method described in the first embodiment is realized.
Embodiment 3
[0162] In one or more embodiments, a computer-readable storage medium is disclosed, on which a computer program is stored. When the program is executed by a processor, the fusion convolutional neural network and channel and space-time described in Embodiment 1 are executed. A method for deep video action recognition with point-of-interest attention models.
PUM
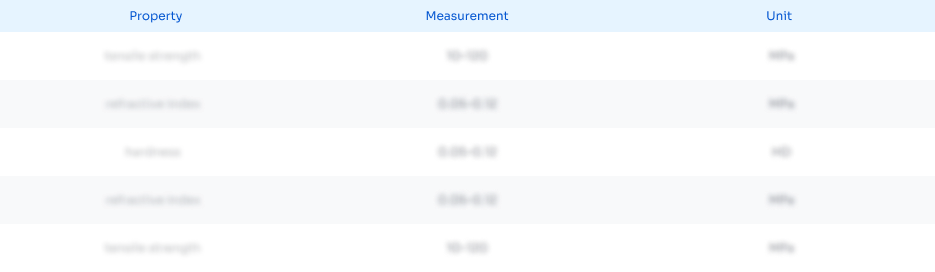
Abstract
Description
Claims
Application Information
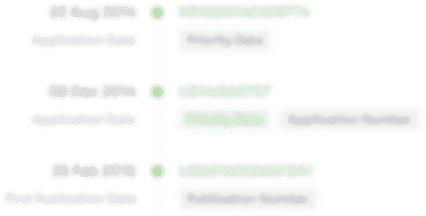
- R&D Engineer
- R&D Manager
- IP Professional
- Industry Leading Data Capabilities
- Powerful AI technology
- Patent DNA Extraction
Browse by: Latest US Patents, China's latest patents, Technical Efficacy Thesaurus, Application Domain, Technology Topic, Popular Technical Reports.
© 2024 PatSnap. All rights reserved.Legal|Privacy policy|Modern Slavery Act Transparency Statement|Sitemap|About US| Contact US: help@patsnap.com