Defect detection method based on deep neural network heat map prediction
A deep neural network and defect detection technology, applied in material defect testing, image enhancement, image analysis, etc., can solve problems such as efficiency affecting production, high requirements, and lack of in-depth integration of technical solution design, to optimize detection speed, The effect of good performance
- Summary
- Abstract
- Description
- Claims
- Application Information
AI Technical Summary
Problems solved by technology
Method used
Image
Examples
Embodiment Construction
[0019] The embodiments of the present invention will be described in detail below with reference to the accompanying drawings, but the present invention can be implemented in many different ways defined and covered by the claims.
[0020] The purpose of the present invention is to solve the practical problems existing in the current industrial surface defect detection, for example: the deep learning model is large in size and the number of parameters is huge, resulting in slow detection speed; on the other hand, the detection of appearance defects in industrial production sites is complicated , specifically manifested in: 1) There are many shapes and types of defects; 2) Users have very strict requirements on defects, and the algorithm is required to accurately detect weak defects and accurately describe the geometry of defects quantitatively; 3) Users have strict requirements on defects. There is no fixed standard for the judgment, and the requirements for the yield rate are f...
PUM
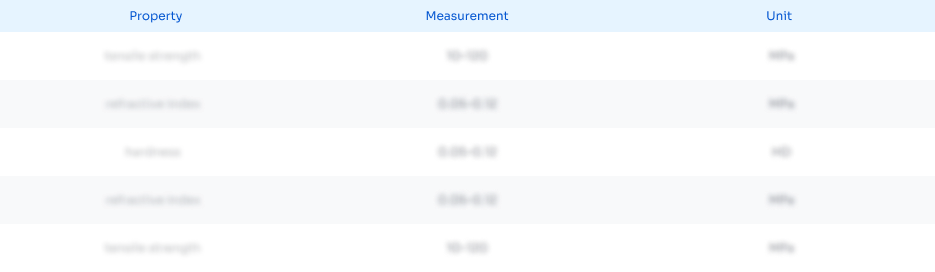
Abstract
Description
Claims
Application Information
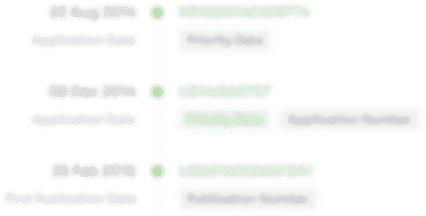
- R&D Engineer
- R&D Manager
- IP Professional
- Industry Leading Data Capabilities
- Powerful AI technology
- Patent DNA Extraction
Browse by: Latest US Patents, China's latest patents, Technical Efficacy Thesaurus, Application Domain, Technology Topic, Popular Technical Reports.
© 2024 PatSnap. All rights reserved.Legal|Privacy policy|Modern Slavery Act Transparency Statement|Sitemap|About US| Contact US: help@patsnap.com