Semi-supervised pedestrian detection method based on deep convolutional network
A technology of pedestrian detection and deep convolution, applied in the field of pedestrian detection, can solve problems such as easy misjudgment, small intra-class difference, false positive class, etc., and achieve the effect of improving anti-noise ability and robustness
- Summary
- Abstract
- Description
- Claims
- Application Information
AI Technical Summary
Problems solved by technology
Method used
Image
Examples
Embodiment
[0028] A semi-supervised pedestrian detection method based on deep convolutional networks, such as figure 2 As shown, the RPN convolutional neural network includes the first five layers of the convolutional network of the VGG-16 network. After the fifth layer of convolution of the VGG-16, a convolution kernel is connected to an intermediate layer convolution with a convolution kernel of 3*3 (candidate window Feature extraction layer), and finally link two 1*1 convolutional layers (output layer), score the target window and predict the relative position of the coordinates, the RPN structure diagram of the input image as an example of 224*224 is as follows figure 1 shown. Specific flow charts, such as figure 1 as shown,
[0029] S1 randomly selects 5% from the 100% complete training set (352 pictures, 1919 pedestrians) of the CUHK-Square dataset as the training set for semi-supervised learning, and retains its labels, which is the labeled training set in this paper (18 pictu...
PUM
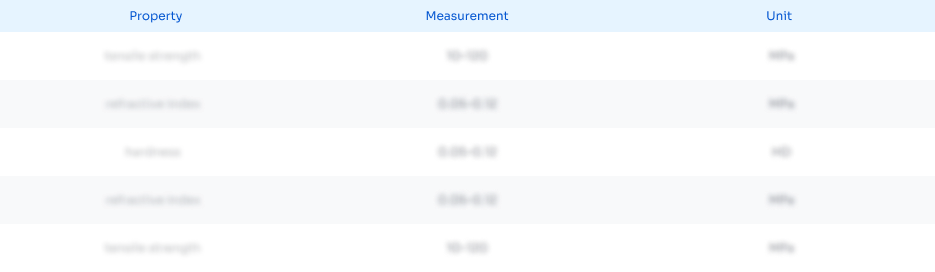
Abstract
Description
Claims
Application Information
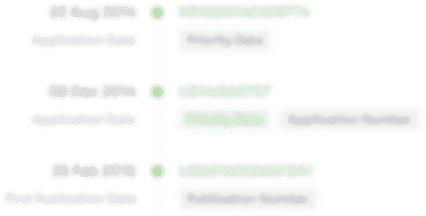
- R&D
- Intellectual Property
- Life Sciences
- Materials
- Tech Scout
- Unparalleled Data Quality
- Higher Quality Content
- 60% Fewer Hallucinations
Browse by: Latest US Patents, China's latest patents, Technical Efficacy Thesaurus, Application Domain, Technology Topic, Popular Technical Reports.
© 2025 PatSnap. All rights reserved.Legal|Privacy policy|Modern Slavery Act Transparency Statement|Sitemap|About US| Contact US: help@patsnap.com