Image fusion method based on joint convolutional self-coding network
A technology of convolutional auto-encoding and image fusion, applied in the field of image fusion based on joint convolutional auto-encoding network, can solve the problems of inability to obtain training label information, insufficient training data for fusion images, etc., to achieve rich information, good quality, high definition effect
- Summary
- Abstract
- Description
- Claims
- Application Information
AI Technical Summary
Problems solved by technology
Method used
Image
Examples
Embodiment Construction
[0055] An embodiment of the present invention ("street" infrared and visible light image) will be described in detail below in conjunction with the accompanying drawings. This embodiment is carried out under the premise of the technical solution of the present invention, as figure 1 As shown, the detailed implementation and specific operation steps are as follows:
[0056] Step 1. During the training process, the image to be fused passes through the private feature branch and the public feature branch of the encoding layer to obtain private features and public features respectively. In order to improve the ability of the joint convolutional self-encoding network for image fusion, we introduced the image fusion evaluation indicators MSE, SSIM, entropy and gradient into the loss function, designed a multi-task loss function for network training, and improved the joint convolutional self-encoding. The feature extraction ability of the network.
[0057] Step 2, during the test pr...
PUM
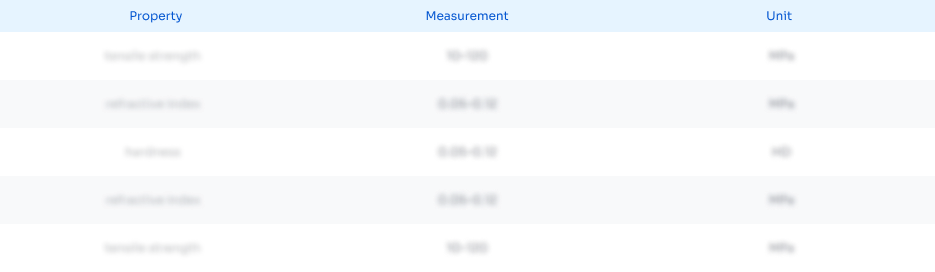
Abstract
Description
Claims
Application Information
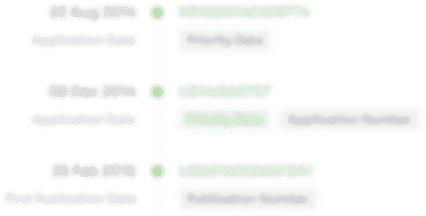
- Generate Ideas
- Intellectual Property
- Life Sciences
- Materials
- Tech Scout
- Unparalleled Data Quality
- Higher Quality Content
- 60% Fewer Hallucinations
Browse by: Latest US Patents, China's latest patents, Technical Efficacy Thesaurus, Application Domain, Technology Topic, Popular Technical Reports.
© 2025 PatSnap. All rights reserved.Legal|Privacy policy|Modern Slavery Act Transparency Statement|Sitemap|About US| Contact US: help@patsnap.com