Virtual sample generation method based on kernel density estimation and Copula function
A technology of kernel density estimation and virtual samples, which is applied in the field of machine learning, can solve problems such as classifier performance degradation and unbalanced classification, and achieve the effects of improving classification effects, generalization capabilities, and improving the distribution of different types of data
- Summary
- Abstract
- Description
- Claims
- Application Information
AI Technical Summary
Problems solved by technology
Method used
Image
Examples
Embodiment 1
[0054] The core requirement of unbalanced data classification is how to improve the classification effect of minority samples. At present, the methods to solve the problem of unbalanced data classification can be roughly divided into data-level methods, algorithm-level methods, and feature selection methods. The processing method at the algorithm level is mainly based on the cost-sensitive learning algorithm, which improves the misclassification cost of a small number of data. Commonly used algorithms include the AdaCost algorithm and the cost-sensitive decision tree classifier. The improvement method based on the algorithm level only simply increases the classification cost of the small-class data, and does not fundamentally improve the classification effect of the minority-class samples. The improvement of the data level has gradually become a mainstream processing method because it can greatly improve the effect of many classifiers on unbalanced data without modifying the cl...
PUM
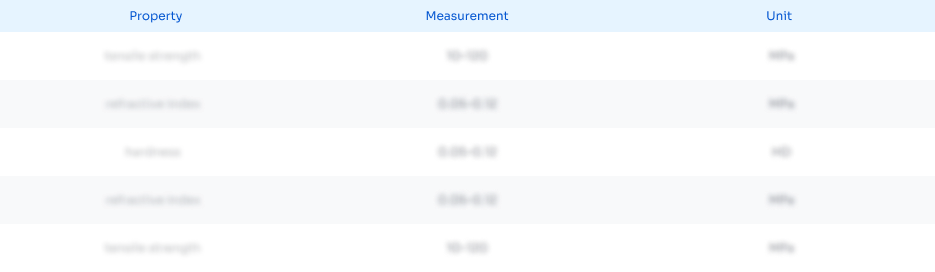
Abstract
Description
Claims
Application Information
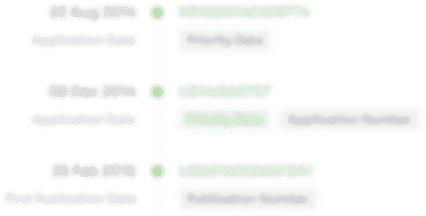
- R&D Engineer
- R&D Manager
- IP Professional
- Industry Leading Data Capabilities
- Powerful AI technology
- Patent DNA Extraction
Browse by: Latest US Patents, China's latest patents, Technical Efficacy Thesaurus, Application Domain, Technology Topic.
© 2024 PatSnap. All rights reserved.Legal|Privacy policy|Modern Slavery Act Transparency Statement|Sitemap