Super-resolution image reconstruction method based on characteristic channel adaptive weighting
A super-resolution image and self-adaptive weighting technology, which is applied in image data processing, graphics and image conversion, neural learning methods, etc., can solve problems affecting image quality and achieve high image peak signal-to-noise ratio
- Summary
- Abstract
- Description
- Claims
- Application Information
AI Technical Summary
Problems solved by technology
Method used
Image
Examples
Embodiment Construction
[0025] The present invention will be described in further detail below in conjunction with the accompanying drawings.
[0026] The specific implementation steps of the super-resolution image reconstruction technology proposed by the present invention are as follows:
[0027] Step 1, improving the existing SE structure to obtain the ISE module.
[0028] Such as figure 1 As shown, on the basis of the original SE network, the input layer and the output layer are residually connected using the following formula to realize adaptive learning of network channel weights.
[0029] E(y)=(σ(W 2 δ(W 1 y))×0.8+σ(y)×0.2)×2
[0030] Among them, y is the input vector of the SE network, E(y) is the channel weight of the network, σ and δ represent the Sigmoid function and the ReLU function respectively, W 1 and W 2 is the weight of the single hidden layer neural network and C is the number of channels.
[0031] Step 2, Simplify the EDSR network.
[0032] The simplified EDSR network mo...
PUM
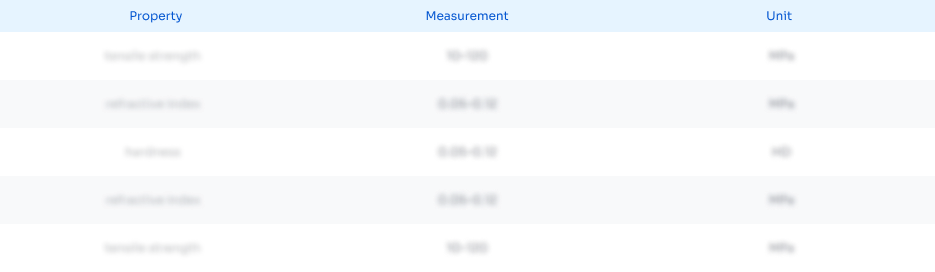
Abstract
Description
Claims
Application Information
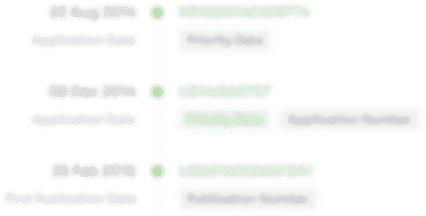
- R&D Engineer
- R&D Manager
- IP Professional
- Industry Leading Data Capabilities
- Powerful AI technology
- Patent DNA Extraction
Browse by: Latest US Patents, China's latest patents, Technical Efficacy Thesaurus, Application Domain, Technology Topic.
© 2024 PatSnap. All rights reserved.Legal|Privacy policy|Modern Slavery Act Transparency Statement|Sitemap