Remote sensing image cloud detection method based on multi-scale fusion semantic segmentation network
A multi-scale fusion, remote sensing image technology, applied in biological neural network models, instruments, character and pattern recognition, etc., can solve the problem of low cloud detection accuracy, achieve fine segmentation, improve cloud detection accuracy, and reduce cloud area detection errors. Effect
- Summary
- Abstract
- Description
- Claims
- Application Information
AI Technical Summary
Problems solved by technology
Method used
Image
Examples
specific Embodiment approach 1
[0038] Specific implementation mode one: as figure 1 As shown, the remote sensing image cloud detection method based on multi-scale fusion semantic segmentation network described in this embodiment comprises the following steps:
[0039] Step 1. Randomly select N from the real panchromatic visible light remote sensing image data set 0 Zhang as the original remote sensing image;
[0040] to N 0 The original remote sensing images are preprocessed to obtain N 0 A preprocessed remote sensing image;
[0041] The data set used in step 1 is: the 2-meter resolution real panchromatic visible light remote sensing image data set taken by Gaofen-1 satellite;
[0042] Step two, put N 0 A preprocessed remote sensing image is used as a training set and input to a weighted multi-scale fusion network (WMSFNet) for training. During the training process, the convolution kernel parameters of the convolutional layer in the semantic segmentation network are continuously updated until the set m...
specific Embodiment approach 2
[0066] Specific embodiment two: the difference between this embodiment and specific embodiment one is: the pair N 0 The original remote sensing images are preprocessed to obtain N 0 A preprocessed remote sensing image, the specific process is as follows:
[0067] For any original remote sensing image, calculate the mean value M of the grayscale of each channel of the original remote sensing image, and then use the grayscale of each pixel in the original remote sensing image to subtract the mean value M to obtain the original remote sensing image The corresponding preprocessed remote sensing image, that is, the gray value of each pixel in the preprocessed remote sensing image corresponding to the original remote sensing image:
[0068] O'(i,j)=O(i,j)-M (1)
[0069] Among them: O(i,j) is the gray value of the pixel point (i,j) in the original remote sensing image, and O′(i,j) is the pixel in the preprocessed remote sensing image corresponding to the original remote sensing ima...
specific Embodiment approach 3
[0071] Specific implementation mode three: as figure 2 and image 3 As shown, the difference between this embodiment and the second embodiment is that the specific process of the second step is:
[0072] Will N 0 A preprocessed remote sensing image is used as a training set to input the semantic segmentation network. Before starting the training, the network parameters of the semantic segmentation network need to be initialized, and the training process can be started after the initialization of the network parameters is completed;
[0073] The semantic segmentation network includes 15 convolutional layers, 5 pooling layers, 2 deconvolution layers and 2 clipping layers, which are respectively:
[0074] Two convolution kernels with a size of 3*3 and a convolution layer with a number of convolution kernels of 64;
[0075] A pooling layer with a convolution kernel size of 2*2 and a number of convolution kernels of 64;
[0076] Two convolution kernels with a size of 3*3 and a...
PUM
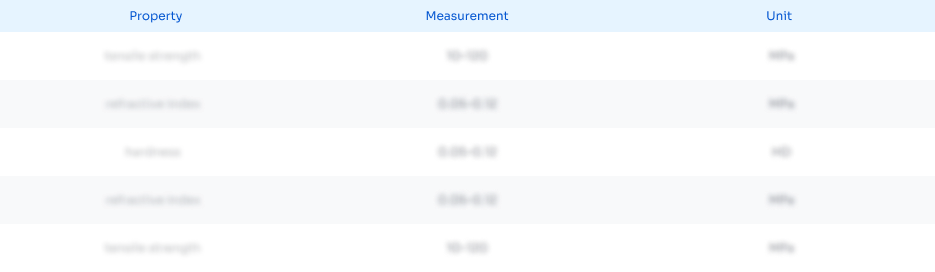
Abstract
Description
Claims
Application Information
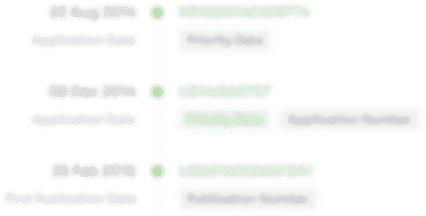
- R&D
- Intellectual Property
- Life Sciences
- Materials
- Tech Scout
- Unparalleled Data Quality
- Higher Quality Content
- 60% Fewer Hallucinations
Browse by: Latest US Patents, China's latest patents, Technical Efficacy Thesaurus, Application Domain, Technology Topic, Popular Technical Reports.
© 2025 PatSnap. All rights reserved.Legal|Privacy policy|Modern Slavery Act Transparency Statement|Sitemap|About US| Contact US: help@patsnap.com