Single image super-resolution reconstruction method based on conditional generative adversarial network
A super-resolution reconstruction and conditional generation technology, applied in the field of image processing, can solve problems such as training collapse, uncontrollable GAN, poor detail effect, etc., and achieve the effect of improving discrimination accuracy
- Summary
- Abstract
- Description
- Claims
- Application Information
AI Technical Summary
Problems solved by technology
Method used
Image
Examples
Embodiment 1
[0056] Such as figure 1 As shown, a single image super-resolution learning method based on conditional generative confrontation network of this embodiment includes the following steps:
[0057] Step 1: The training set is composed of high-resolution images and low-resolution images obtained by downsampling the high-resolution images; specifically:
[0058] Using the 16,700 high-resolution training images of the VOC2012 training set, the high-resolution training images are down-sampled by 4 times using the bicubic interpolation method to obtain low-resolution images. Then use the random cropping method to randomly crop a high-resolution image block of size 88x88 for each high-resolution training image, and crop an image block of size 22x22 in the corresponding low-resolution image at the same position. In this way, training image blocks of corresponding proportions are obtained, and finally high and low resolution training image blocks are obtained;
[0059] The random cropp...
Embodiment 2
[0094] The single image super-resolution learning method based on the conditional generation confrontation network of the present embodiment includes the following steps:
[0095] Step 1: Downsample the high-resolution training image to obtain a low-resolution training image. Specifically: the VOC2012 training set is adopted, and of course the present invention is also applicable to other training sets. The algorithm used for the low-resolution images obtained by downsampling the high-resolution training images is the bicubic interpolation algorithm. Second, corresponding random cropping of high and low resolution images is required. The crop size can be set, but the high-resolution tiles are 4 times the size of the low-resolution tiles. Cropping is to randomly crop an image patch on each image. This embodiment is also suitable for randomly cropping multiple image blocks for training;
[0096] Step 2: Downsampling the images in the test set to obtain low-resolution test im...
PUM
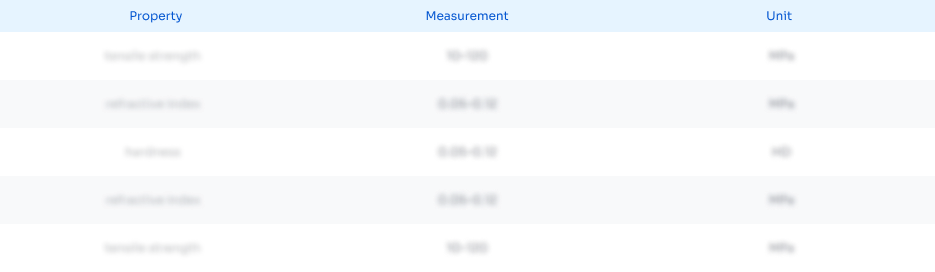
Abstract
Description
Claims
Application Information
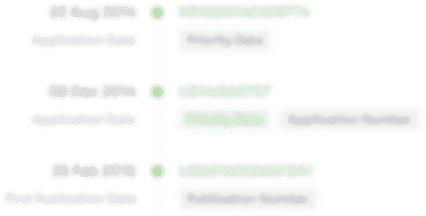
- R&D Engineer
- R&D Manager
- IP Professional
- Industry Leading Data Capabilities
- Powerful AI technology
- Patent DNA Extraction
Browse by: Latest US Patents, China's latest patents, Technical Efficacy Thesaurus, Application Domain, Technology Topic, Popular Technical Reports.
© 2024 PatSnap. All rights reserved.Legal|Privacy policy|Modern Slavery Act Transparency Statement|Sitemap|About US| Contact US: help@patsnap.com