SAR image target classification method based on deep convolutional neural network
A deep convolution and neural network technology, applied in the field of radar, can solve the problems of low target classification accuracy, low classification accuracy, and background clutter removal in SAR images, and achieve the effect of suppressing automatic extraction, retaining shape features, and improving accuracy.
- Summary
- Abstract
- Description
- Claims
- Application Information
AI Technical Summary
Problems solved by technology
Method used
Image
Examples
Embodiment Construction
[0043] The present invention will be further described in detail below in conjunction with the drawings and specific embodiments.
[0044] Reference figure 1 , A SAR image target classification method based on deep convolutional neural network, including the following steps:
[0045] Step 1) Obtain the training sample set R 0 And test sample set E 0 :
[0046] Step 1a) This embodiment uses the MSTAR data set including ten types of ground vehicle targets with pitch angles of 15° and 17°: armored vehicles: BMP-2, BRDM-2, BTR-60, BTR-70; tanks: T62, T72 ; Rocket launcher: 2S1; air defense unit: ZSU-234; truck: ZIL-131; bulldozer: D7. In the experiment, 3671 SAR images containing the 10 types of ground vehicle targets and a size of 128×128 under a 17° pitch angle are selected as the training sample set R 0 ; Select 3203 SAR images containing the 10 types of ground vehicle targets and the size of 128×128 at a pitch angle of 15° as the test sample set E 0 , The composition of each type of...
PUM
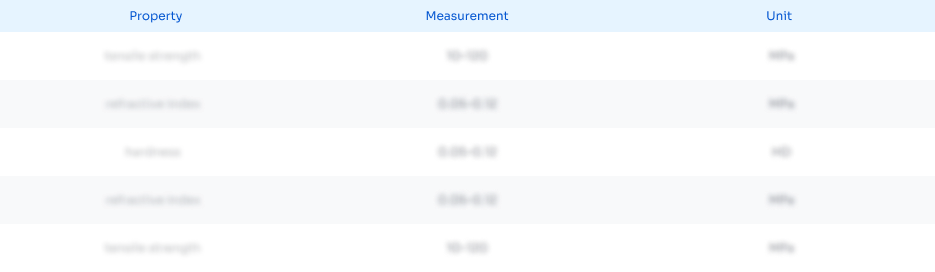
Abstract
Description
Claims
Application Information
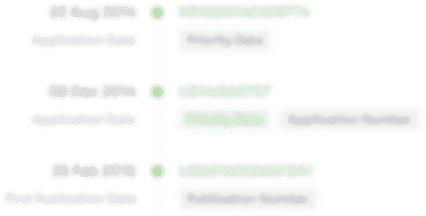
- R&D
- Intellectual Property
- Life Sciences
- Materials
- Tech Scout
- Unparalleled Data Quality
- Higher Quality Content
- 60% Fewer Hallucinations
Browse by: Latest US Patents, China's latest patents, Technical Efficacy Thesaurus, Application Domain, Technology Topic, Popular Technical Reports.
© 2025 PatSnap. All rights reserved.Legal|Privacy policy|Modern Slavery Act Transparency Statement|Sitemap|About US| Contact US: help@patsnap.com