Concrete crack identification method based on YOLOv3 deep learning
A crack identification and deep learning technology, applied in the field of concrete structure damage detection, can solve the problems of low detection efficiency, high cost, and high computing cost, and achieve the effect of reducing computing cost, high accuracy, and simplifying complexity
- Summary
- Abstract
- Description
- Claims
- Application Information
AI Technical Summary
Problems solved by technology
Method used
Image
Examples
Embodiment Construction
[0052] In order to make the object, technical solution and advantages of the present invention more clear, the present invention will be further described in detail below in conjunction with the examples. It should be understood that the specific embodiments described here are only used to explain the present invention, not to limit the present invention.
[0053] For the currently commonly used deep learning methods similar to exhaustive, a large amount of data is required, lack of pertinence, high computing costs, and low detection efficiency. The invention is a crack detection technology with strong robustness, good generalization ability, high detection efficiency and accuracy, originality and more suitable for engineering field.
[0054] The application principle of the present invention will be described in detail below in conjunction with the accompanying drawings.
[0055] Such as figure 1 As shown, the concrete crack identification method based on YOLOv3 deep learn...
PUM
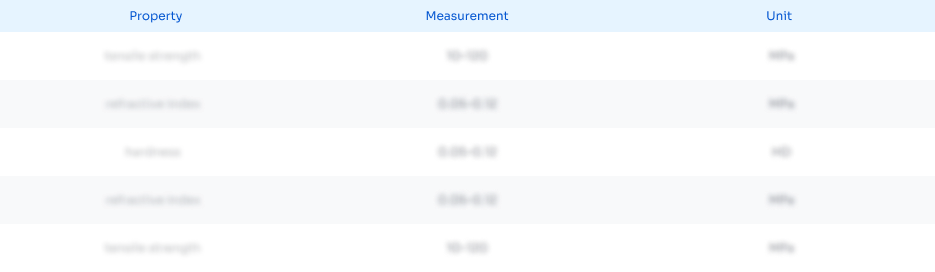
Abstract
Description
Claims
Application Information
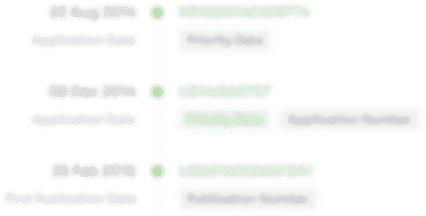
- R&D Engineer
- R&D Manager
- IP Professional
- Industry Leading Data Capabilities
- Powerful AI technology
- Patent DNA Extraction
Browse by: Latest US Patents, China's latest patents, Technical Efficacy Thesaurus, Application Domain, Technology Topic, Popular Technical Reports.
© 2024 PatSnap. All rights reserved.Legal|Privacy policy|Modern Slavery Act Transparency Statement|Sitemap|About US| Contact US: help@patsnap.com