Data volume sculptor for deep learning acceleration
A data body and accelerator technology, applied in image data processing, neural learning methods, electrical digital data processing, etc., can solve problems such as weakening performance
- Summary
- Abstract
- Description
- Claims
- Application Information
AI Technical Summary
Problems solved by technology
Method used
Image
Examples
Embodiment Construction
[0108] The present invention can be understood more easily by referring to the following detailed description of preferred embodiments of the invention. It is to be understood that the terminology used herein is for the purpose of describing particular embodiments only and is not intended to be limiting. It should also be understood that unless specifically defined herein, terms used herein are to be given their conventional meanings as known in the relevant art.
[0109] Deep convolution processing in neural networks is known to produce excellent results when performing actions such as object classification in graphics. Less developed, however, is the process of efficiently detecting and classifying objects, scenes, actions, or other points of interest in video streams. Because video data is complex, and because videos lack annotations that can be so easily attached to image data, means for detecting points of interest within videos have not received as much attention. Wher...
PUM
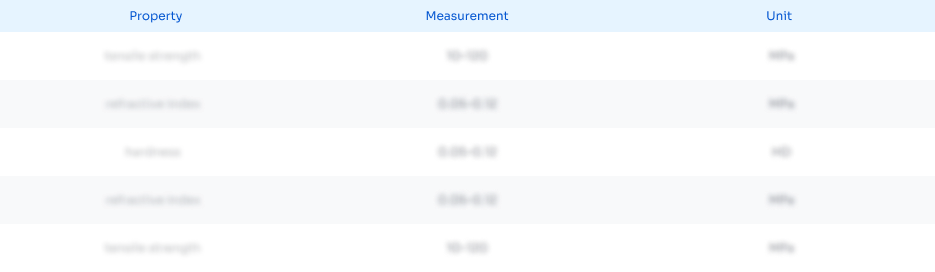
Abstract
Description
Claims
Application Information
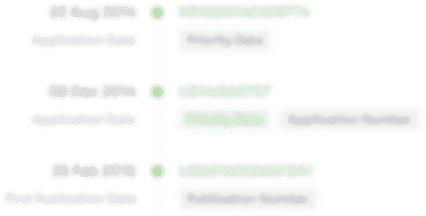
- R&D
- Intellectual Property
- Life Sciences
- Materials
- Tech Scout
- Unparalleled Data Quality
- Higher Quality Content
- 60% Fewer Hallucinations
Browse by: Latest US Patents, China's latest patents, Technical Efficacy Thesaurus, Application Domain, Technology Topic, Popular Technical Reports.
© 2025 PatSnap. All rights reserved.Legal|Privacy policy|Modern Slavery Act Transparency Statement|Sitemap|About US| Contact US: help@patsnap.com