Latent semantic learning method for multi-view multi-label data
A learning method and multi-label technology, applied in the field of latent semantic learning for multi-view and multi-label data, can solve problems such as low-dimensional latent semantic space, achieve the effect of overcoming shortcomings and limitations, and solving the problem of multi-view complementarity
- Summary
- Abstract
- Description
- Claims
- Application Information
AI Technical Summary
Problems solved by technology
Method used
Image
Examples
Embodiment 1
[0046] Such as figure 1 As shown, the implementation of the present invention provides a hidden semantic space learning method that fuses multi-view and multi-label information, including:
[0047]Read the multi-view and multi-label information, and then learn the projection matrix of the multi-view information. The present invention involves the learning of multiple views, which contains multiple sets of variables that need to be updated. At the same time, the model has orthogonal constraints on the learned latent semantic space. Therefore, alternate direction multiplier method and bregman iteration are introduced to optimize the solution. ADMM adopts the method of decomposing coordinates to solve the optimization problem into smaller local sub-problems, and then the solutions of these local sub-problems are used to restore the solution of the original large-scale optimization problem in a coordinated manner. For each view-related variable update, stop updating when the erro...
Embodiment 2
[0049] The hidden semantic space learning method for fusing multi-view and multi-label information provided in this embodiment is the same as that in Embodiment 1, and only the steps in the method are further limited.
[0050] Step S1: Data preprocessing, read multi-view and multi-label data for preprocessing, the preprocessing part removes the stop words of the text data and vectorizes the data through the TF-IDF algorithm, and uses the feature vectorization result obtained from the preprocessing as the method input of;
[0051] Step S2: Build a latent semantic learning model for multi-view and multi-label data; based on the principle of mapping data to low-dimensional space to minimize reconstruction errors and retain as much information as possible, a classifier that integrates different perspectives and characteristics is constructed, and at the same time Combined with the correlation of multiple tags, the present invention optimizes the following constrained objective fun...
PUM
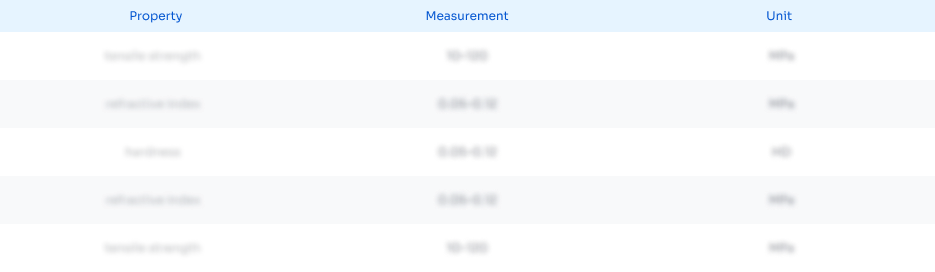
Abstract
Description
Claims
Application Information
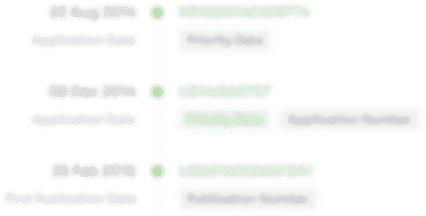
- R&D
- Intellectual Property
- Life Sciences
- Materials
- Tech Scout
- Unparalleled Data Quality
- Higher Quality Content
- 60% Fewer Hallucinations
Browse by: Latest US Patents, China's latest patents, Technical Efficacy Thesaurus, Application Domain, Technology Topic, Popular Technical Reports.
© 2025 PatSnap. All rights reserved.Legal|Privacy policy|Modern Slavery Act Transparency Statement|Sitemap|About US| Contact US: help@patsnap.com