Image sentiment analysis method based on multi-task learning mode
A multi-task learning and sentiment analysis technology, applied in the field of image sentiment analysis based on multi-task learning, can solve the huge semantic gap and other problems
- Summary
- Abstract
- Description
- Claims
- Application Information
AI Technical Summary
Problems solved by technology
Method used
Image
Examples
Embodiment Construction
[0058] The present invention will be further described below in combination with specific embodiments. Wherein, the accompanying drawings are only for illustrative purposes, and represent only schematic diagrams rather than physical diagrams, and should not be construed as limitations on this patent.
[0059] An image sentiment analysis method based on a multi-task learning method provided by the present invention, the overall structure of the specific implementation is shown as follows figure 1 As shown, the method flow diagram is as follows figure 2 shown, including the following steps:
[0060] S1: Build an image emotional attribute detector and an image emotional label classifier;
[0061] The structure of the image emotion attribute detector is as follows: image 3 As shown, the designed structure includes a front-end VGG-16 network convolution layer, a back-end fully connected layer, and a softmax layer; in this embodiment, the front-end borrows [K.Simonyan and A.Zis...
PUM
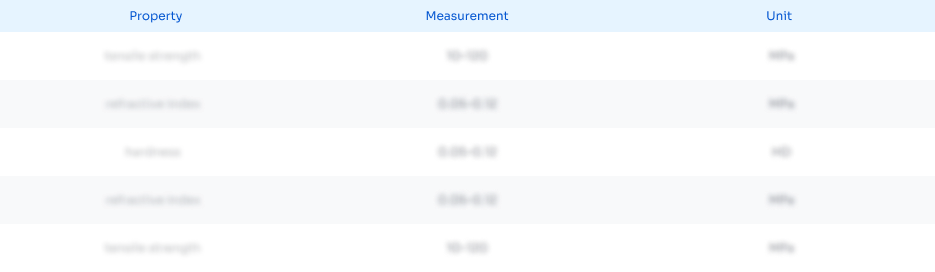
Abstract
Description
Claims
Application Information
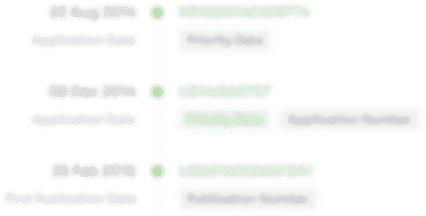
- R&D Engineer
- R&D Manager
- IP Professional
- Industry Leading Data Capabilities
- Powerful AI technology
- Patent DNA Extraction
Browse by: Latest US Patents, China's latest patents, Technical Efficacy Thesaurus, Application Domain, Technology Topic, Popular Technical Reports.
© 2024 PatSnap. All rights reserved.Legal|Privacy policy|Modern Slavery Act Transparency Statement|Sitemap|About US| Contact US: help@patsnap.com