City large-scale road network traffic speed prediction method based on deep integrated learning
An integrated learning and speed prediction technology, applied in neural learning methods, traffic flow detection, traffic control systems for road vehicles, etc., to achieve the effect of improving prediction accuracy, strong periodicity, and good spatial scalability
- Summary
- Abstract
- Description
- Claims
- Application Information
AI Technical Summary
Problems solved by technology
Method used
Image
Examples
Embodiment Construction
[0020] This invention is based on the research of National Key Research and Development Project (2018YFB1600904), National Natural Science Foundation Project (71771198, 71961137005) and Zhejiang Provincial Natural Science Foundation Outstanding Youth Project (LR17E080002), and involves a large-scale urban road network based on deep integrated learning The traffic speed prediction method, the present invention will be further described below in conjunction with specific embodiments, but the protection scope of the present invention is not limited thereto.
[0021] Taking the second, third, fourth ring roads and their radiation roads in Beijing as an example, there are 308 detectors in total, the road network covers an area of about 300 square kilometers, and the total road length is about 360 kilometers. The topological structure of the road section and the layout position of the detector are as follows: figure 1 shown. The short-term prediction of the traffic speed of the r...
PUM
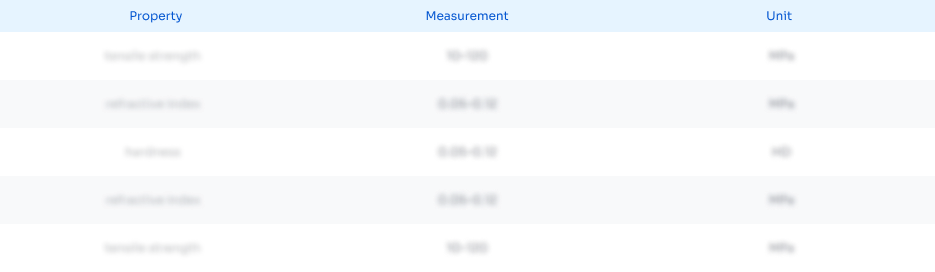
Abstract
Description
Claims
Application Information
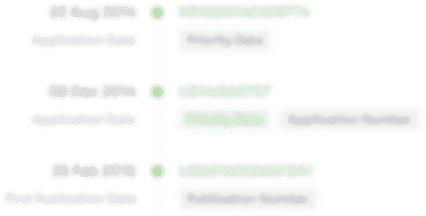
- R&D Engineer
- R&D Manager
- IP Professional
- Industry Leading Data Capabilities
- Powerful AI technology
- Patent DNA Extraction
Browse by: Latest US Patents, China's latest patents, Technical Efficacy Thesaurus, Application Domain, Technology Topic, Popular Technical Reports.
© 2024 PatSnap. All rights reserved.Legal|Privacy policy|Modern Slavery Act Transparency Statement|Sitemap|About US| Contact US: help@patsnap.com