Deep learning network intrusion detection method based on improved learning rate
A deep learning network and network intrusion detection technology, applied in data exchange network, digital transmission system, electrical components, etc., can solve problems such as slow convergence speed, difficult model training, and reduced accuracy, so as to improve detection efficiency and recognition accuracy The effect of rate and fast convergence
- Summary
- Abstract
- Description
- Claims
- Application Information
AI Technical Summary
Problems solved by technology
Method used
Image
Examples
Embodiment Construction
[0037] The present invention will be described in further detail below in conjunction with the examples, but the protection scope of the present invention is not limited thereto.
[0038] Step 1: Improve the method for determining the learning rate and the number of iterations of the deep belief network (DBN) model in training.
[0039]In the present invention, in the traditional algorithm, the learning rate ε needs to be manually adjusted to an appropriate value for both error control and convergence speed. The disadvantage of this is that the entire model needs to be continuously adjusted manually during the training process. If the learning rate is too large, the reconstruction error will increase; if the learning rate is too small, the above problems can be avoided, but the training convergence speed will be slower.
[0040] Step 1 of the present invention includes the following steps.
[0041] Step 1.1: Let ε ij weight W for each connection ij The corresponding learnin...
PUM
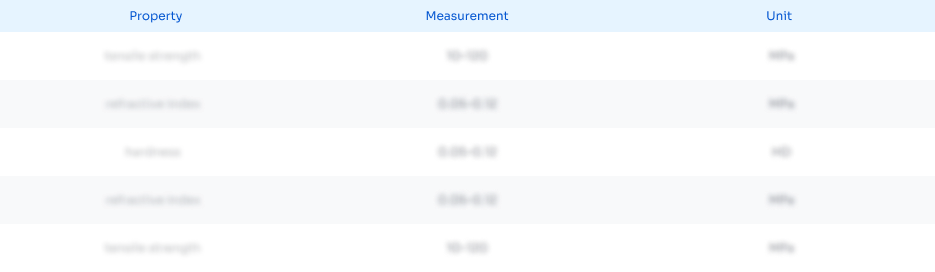
Abstract
Description
Claims
Application Information
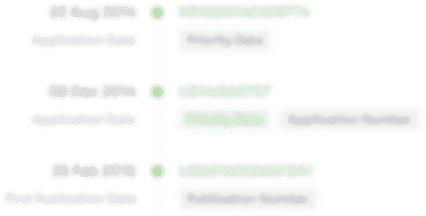
- R&D Engineer
- R&D Manager
- IP Professional
- Industry Leading Data Capabilities
- Powerful AI technology
- Patent DNA Extraction
Browse by: Latest US Patents, China's latest patents, Technical Efficacy Thesaurus, Application Domain, Technology Topic, Popular Technical Reports.
© 2024 PatSnap. All rights reserved.Legal|Privacy policy|Modern Slavery Act Transparency Statement|Sitemap|About US| Contact US: help@patsnap.com