CEEMDAN-based financial time series combination prediction method
A financial time series and combined forecasting technology, applied in finance, reservations, instruments, etc., can solve the problems of difficult financial data forecasting and forecasting accuracy, cumbersome forecasting method process, etc., to achieve good adaptability, good performance, and accurate forecasting effect. Effect
- Summary
- Abstract
- Description
- Claims
- Application Information
AI Technical Summary
Problems solved by technology
Method used
Image
Examples
Embodiment Construction
[0010] The present invention will be further described in detail below in combination with specific embodiments.
[0011] 1. Through the CEEMDAN algorithm, the original financial time series Decomposed into several IMF subsequences and a remainder , and then divide all subsequences into test set and training set.
[0012] 2. Predictive model construction;
[0013] 1) Use LSTM unit to build a reliable time series prediction network, input IMF subsequence
[0014] The network consists of two layers of LSTM units and a layer of fully connected network. The abstract pattern in the time series is learned by using the two-layer LSTM network, which is the core part of the network. The structure of the network is as figure 2 As shown, since the output of the LSTM unit is a multidimensional vector, the output of the last LSTM unit of the second layer (layer2) network is connected to the fully connected network of the third layer (layer3). During the training process, the para...
PUM
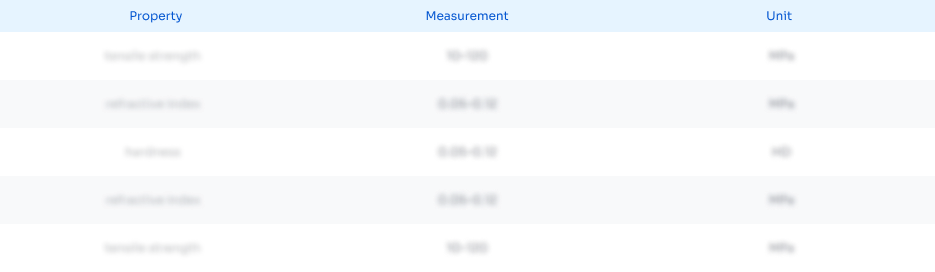
Abstract
Description
Claims
Application Information
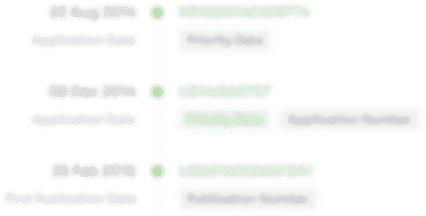
- R&D Engineer
- R&D Manager
- IP Professional
- Industry Leading Data Capabilities
- Powerful AI technology
- Patent DNA Extraction
Browse by: Latest US Patents, China's latest patents, Technical Efficacy Thesaurus, Application Domain, Technology Topic, Popular Technical Reports.
© 2024 PatSnap. All rights reserved.Legal|Privacy policy|Modern Slavery Act Transparency Statement|Sitemap|About US| Contact US: help@patsnap.com