Vegetation classification method based on machine learning algorithm and multi-source remote sensing data fusion
A technology of machine learning and remote sensing data, applied in machine learning, neural learning methods, instruments, etc., can solve problems such as poor applicability and low accuracy, and achieve the effects of low equipment cost, complete parameter indicators, and strong operability
- Summary
- Abstract
- Description
- Claims
- Application Information
AI Technical Summary
Problems solved by technology
Method used
Image
Examples
Embodiment
[0036] The method of the present invention will be further described below with reference to the accompanying drawings and embodiments. The embodiments are only to help readers better understand the method of the present invention, and are not intended to limit the protection scope of the claims of the present invention.
[0037] The methods provided by the embodiments based on multi-source remote sensing data fusion and object-oriented classification greatly improve the ability of accurate, efficient, quantitative acquisition, analysis, calculation and processing of different vegetation monitoring index data in the monitoring of terrestrial plant ecological environment. Revolutionary changes in the display effect and method of monitoring results. It fills in the technical gaps in the ecological environment monitoring work that there are no conventional monitoring methods and means for the quantitative monitoring of vegetation, and greatly improves the automation of field monitori...
PUM
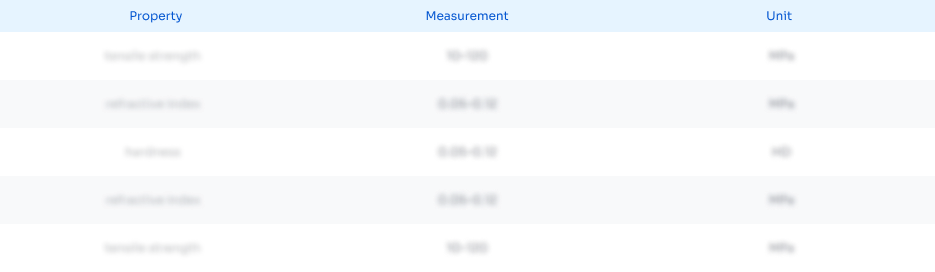
Abstract
Description
Claims
Application Information
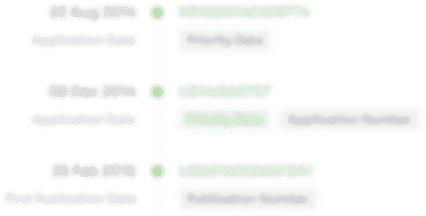
- R&D Engineer
- R&D Manager
- IP Professional
- Industry Leading Data Capabilities
- Powerful AI technology
- Patent DNA Extraction
Browse by: Latest US Patents, China's latest patents, Technical Efficacy Thesaurus, Application Domain, Technology Topic.
© 2024 PatSnap. All rights reserved.Legal|Privacy policy|Modern Slavery Act Transparency Statement|Sitemap