Time sequence physiological data classification method and device, storage medium and processor
A technology of physiological data and classification method, applied in the field of data processing, can solve the problems of difficult to describe the internal pattern of big data, poor performance, weak generalization ability, etc., to avoid expert bias and information loss, efficient calculation, and broad receptive field. effect of size
- Summary
- Abstract
- Description
- Claims
- Application Information
AI Technical Summary
Problems solved by technology
Method used
Image
Examples
Embodiment 1
[0057] Such as Figure 3-7 As shown, this embodiment provides a time-series physiological data classification method, which is based on deep learning and can realize end-to-end decision output, mainly including four stages of data preprocessing, model construction, offline training and online decision-making.
[0058] 1. Data preprocessing
[0059] see Figure 4 , before model training, multi-source sign data and corresponding annotations are extracted from the original medical database, and then data preprocessing is performed on the data. The first step of data preprocessing is data normalization. Since the time-series physiological data are collected from different organs and the collection equipment is different, the signal-to-noise ratio in the original data will be different. For example, the data collected by non-contact equipment will cause large fluctuations in the amplitude of the collected signals due to the difference in the patient's posture (sideways and uprigh...
Embodiment 2
[0081] Corresponding to the time-series physiological data classification method detailed in Embodiment 1, this embodiment provides a time-series physiological data classification device, including:
[0082] The original data acquisition and preprocessing module is used to extract multi-source sign data from the database, divide the data into training data and test data, and perform data preprocessing;
[0083] The model construction module is used to build the deep learning model DeepPhysioNet. The DeepPhysioNet model adopts the neural network architecture of the encoder-decoder. The head of the encoder is a basic feature learning unit composed of a convolutional neural network, and then connected by skipping words. Constitute a sequence residual unit to deepen the network and avoid the problem of gradient degradation in the network. Finally, the attention mechanism is introduced by the representation learning unit. The decoder automatically extracts powerful features from the...
Embodiment 3
[0096] This embodiment provides a storage medium on which a computer program is stored, and when the program is running, the time-series physiological data classification method described in Embodiment 1 is executed.
PUM
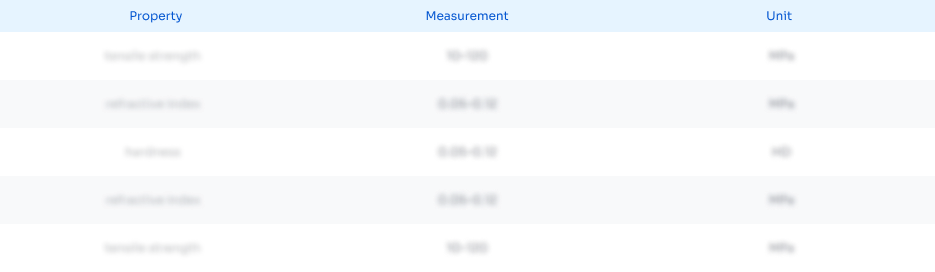
Abstract
Description
Claims
Application Information
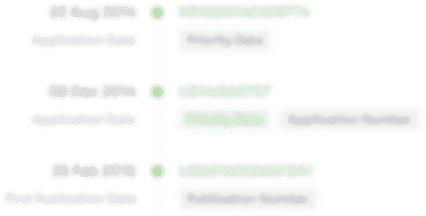
- Generate Ideas
- Intellectual Property
- Life Sciences
- Materials
- Tech Scout
- Unparalleled Data Quality
- Higher Quality Content
- 60% Fewer Hallucinations
Browse by: Latest US Patents, China's latest patents, Technical Efficacy Thesaurus, Application Domain, Technology Topic, Popular Technical Reports.
© 2025 PatSnap. All rights reserved.Legal|Privacy policy|Modern Slavery Act Transparency Statement|Sitemap|About US| Contact US: help@patsnap.com