Mechanical part fault diagnosis method based on deep learning under data imbalance
A technology for mechanical parts and fault diagnosis, applied in computer parts, neural learning methods, instruments, etc., can solve problems such as weak generalization ability, lack of theoretical basis, inability to expand data characteristics, etc., to enhance generalization ability , the effect of balanced fault data, good classification accuracy
- Summary
- Abstract
- Description
- Claims
- Application Information
AI Technical Summary
Problems solved by technology
Method used
Image
Examples
Embodiment Construction
[0034] The present invention will be described in further detail below in conjunction with the accompanying drawings.
[0035] refer to figure 1 , a method for fault diagnosis of mechanical parts based on deep learning under data imbalance, including the following steps:
[0036] 1) Intercept the vibration signals of the mechanical parts acquired by the sensor at equal intervals to form the original sample set Each sample contains 2048 continuous sample points, and then fast Fourier transform is performed on the original sample set to obtain the frequency domain sample data set Each frequency domain sample contains 1024 sample points, that is, real samples, where i represents the i-th type of fault of mechanical parts;
[0037] 2) Input the frequency domain sample data obtained in step 1) into WGAN for confrontation training, figure 2 It is a schematic diagram of the structure of WGAN. Compared with native GAN, it solves the problems of gradient disappearance and model c...
PUM
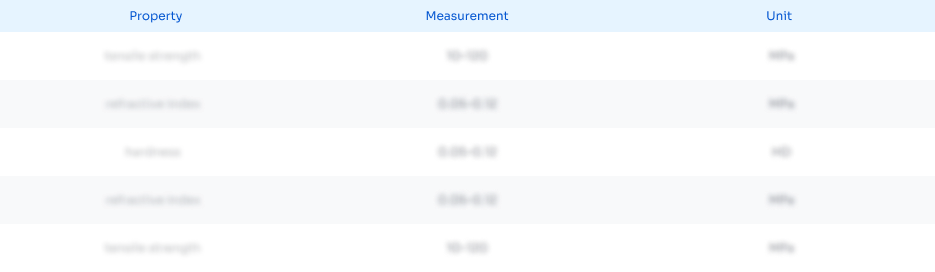
Abstract
Description
Claims
Application Information
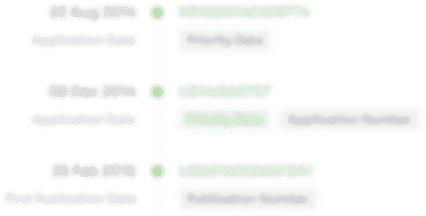
- R&D
- Intellectual Property
- Life Sciences
- Materials
- Tech Scout
- Unparalleled Data Quality
- Higher Quality Content
- 60% Fewer Hallucinations
Browse by: Latest US Patents, China's latest patents, Technical Efficacy Thesaurus, Application Domain, Technology Topic, Popular Technical Reports.
© 2025 PatSnap. All rights reserved.Legal|Privacy policy|Modern Slavery Act Transparency Statement|Sitemap|About US| Contact US: help@patsnap.com