Vehicle image optimization method and system based on adversarial learning
An optimization method and image technology, applied in the field of computer vision, can solve the problems of difficult self-adaptation of complex scenes, factors that reduce the recognition accuracy are not taken into account, manual extraction, etc., to achieve a simple, efficient and good image optimization process. Scenario adaptability and robustness, the effect of reducing deployment difficulty and cost
- Summary
- Abstract
- Description
- Claims
- Application Information
AI Technical Summary
Problems solved by technology
Method used
Image
Examples
Embodiment
[0048] Such as figure 1 , figure 2 As shown, this embodiment provides a vehicle image optimization method based on adversarial learning. Firstly, vehicle images from different angles are collected, various scenes are simulated through image transformation operations, and then a generative adversarial network model is constructed. The scene is divided into pairs to train the model, and finally the generator is reserved as the final vehicle image optimization model. The specific steps are as follows:
[0049] S1: Collect vehicle images taken from different angles, and divide the images into two types: centered vehicle angle and non-centered vehicle angle. The centered vehicle angle image is a standard scene image. In the generation confrontation network, the standard scene image is the conversion target, which is optimized for low-quality images. To provide a reference, the standard scene image is also a real high-quality image;
[0050] S2: Preprocess the non-centered vehicl...
PUM
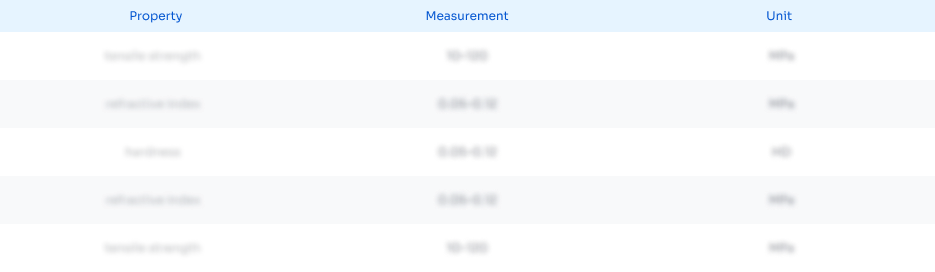
Abstract
Description
Claims
Application Information
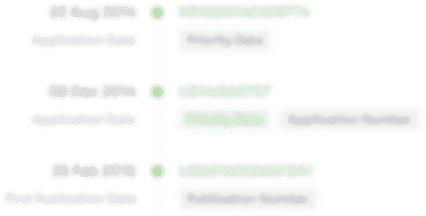
- R&D Engineer
- R&D Manager
- IP Professional
- Industry Leading Data Capabilities
- Powerful AI technology
- Patent DNA Extraction
Browse by: Latest US Patents, China's latest patents, Technical Efficacy Thesaurus, Application Domain, Technology Topic, Popular Technical Reports.
© 2024 PatSnap. All rights reserved.Legal|Privacy policy|Modern Slavery Act Transparency Statement|Sitemap|About US| Contact US: help@patsnap.com