An auxiliary screening method for genetic metabolic diseases based on multi-domain fusion learning
A metabolic disease and screening technology, applied in the field of information technology applications, can solve the problems of difficult screening data, waste of medical resources, reducing the screening accuracy of the threshold method, etc., and achieve the effect of reducing the false positive rate
- Summary
- Abstract
- Description
- Claims
- Application Information
AI Technical Summary
Problems solved by technology
Method used
Image
Examples
Embodiment Construction
[0036] The technical solution of the present invention will be further described below in conjunction with examples.
[0037] The assisted screening method for genetic metabolic diseases based on multi-domain fusion learning of the present invention includes the following two stages:
[0038] The first stage:
[0039] For data from m regions or screening centers, train m neural networks with the same structure but different network parameters;
[0040] In this example, each neural network is set to include four hidden layers, and the number of neurons in each layer is 16, 8, 8, and 4 in sequence; the hidden layer uses ReLU as the activation function, and the output layer uses Sigmoid as the activation function.
[0041] second stage:
[0042] Establish the main neural network. The main neural network includes a frozen layer, a springboard layer, and a specific domain layer; in this example, the main neural network is set to include m*2 frozen layers, 2 springboard layers, an...
PUM
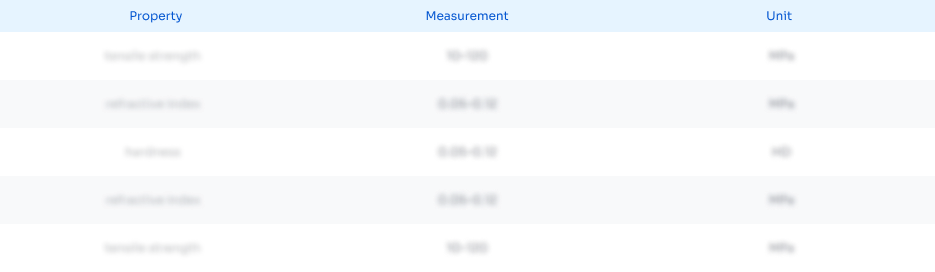
Abstract
Description
Claims
Application Information
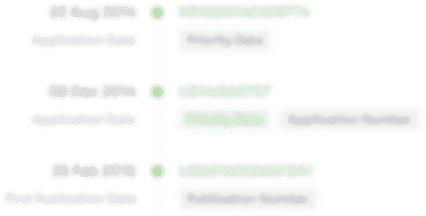
- R&D Engineer
- R&D Manager
- IP Professional
- Industry Leading Data Capabilities
- Powerful AI technology
- Patent DNA Extraction
Browse by: Latest US Patents, China's latest patents, Technical Efficacy Thesaurus, Application Domain, Technology Topic, Popular Technical Reports.
© 2024 PatSnap. All rights reserved.Legal|Privacy policy|Modern Slavery Act Transparency Statement|Sitemap|About US| Contact US: help@patsnap.com