Dynamic multi-level system modeling and state prediction method based on hybrid cognition
A prediction method and technology of system state, applied in the direction of specific mathematical model, prediction, calculation model, etc., can solve the problem of unable to find hidden relationship in network, model unable to predict system state, large deviation, etc.
- Summary
- Abstract
- Description
- Claims
- Application Information
AI Technical Summary
Problems solved by technology
Method used
Image
Examples
Embodiment
[0040] The method of this patent is now described through the application of a certain energy storage battery pack system.
[0041] Step 1, using fault tree and static Bayesian network method to carry out system analysis;
[0042] First, through the fault tree analysis of the energy storage battery pack system, the basic events leading to system faults are obtained. The fault tree is as follows: image 3 shown; then, the fault tree model is transformed into a static Bayesian network structure (DAG) such as Figure 4 As shown, the parameters in the network are given by the fault tree logic relationship and expert experience.
[0043] Step 2. The hybrid cognitive method analyzes a certain energy storage battery pack system, and improves the static Bayesian network formed in step 1.
[0044] Firstly, STAMP analysis is carried out on the energy storage battery system, and the obtained control process model diagram is as follows: Figure 5shown. According to the STAMP analysis ...
PUM
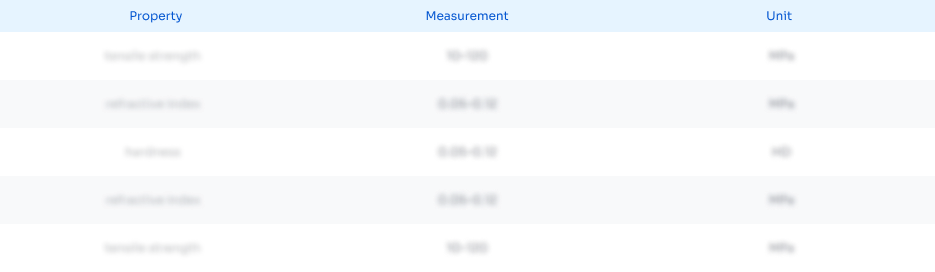
Abstract
Description
Claims
Application Information
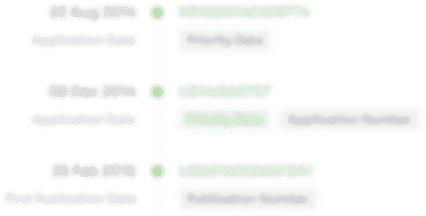
- R&D Engineer
- R&D Manager
- IP Professional
- Industry Leading Data Capabilities
- Powerful AI technology
- Patent DNA Extraction
Browse by: Latest US Patents, China's latest patents, Technical Efficacy Thesaurus, Application Domain, Technology Topic, Popular Technical Reports.
© 2024 PatSnap. All rights reserved.Legal|Privacy policy|Modern Slavery Act Transparency Statement|Sitemap|About US| Contact US: help@patsnap.com