DNN model training method and device
A model training and data set technology, applied in the field of machine learning, can solve the problems of unfavorable business promotion, long process, low prediction accuracy, etc.
- Summary
- Abstract
- Description
- Claims
- Application Information
AI Technical Summary
Problems solved by technology
Method used
Image
Examples
Embodiment 1
[0051] see figure 1 , the present embodiment provides a DNN model training method, comprising: obtaining a first data set and a second data set, respectively performing unified dimension expansion on the dimension vectors in the first data set and the second data set; setting the structure of the DNN model parameters, and initialize the linear relationship coefficient matrix W of each level in the first data set L and bias vector b L ; Use the linear relationship coefficient matrix W corresponding to the second level above L and bias vector b L Convert the data in the first data set and the second data set respectively; convert and superimpose the data in the first data set and the second data set, traverse each element in it, perform forward propagation calculations, and output the results of each neuron in the second level; Based on the results of each neuron in the second level, the forward propagation calculation is performed and the results of each neuron in the third ...
Embodiment 2
[0081] This embodiment provides a DNN model training device, including:
[0082] A data acquisition unit, configured to acquire a first data set and a second data set, and respectively perform unified dimension expansion on the dimension vectors in the first data set and the second data set;
[0083] The initialization unit is used to set the structural parameters of the DNN model, and initialize the linear relationship coefficient matrix W of each level in the first data set L and bias vector b L ;
[0084] A data conversion unit, configured to use the linear relationship coefficient matrix W corresponding to the above-mentioned second level L and bias vector b L respectively converting the data in the first data set and the second data set;
[0085] The first calculation unit is configured to continue traversing the superimposed elements in the next level based on the results of each neuron in the second level, and correspondingly output the results of each neuron in the...
Embodiment 3
[0094] This embodiment provides a computer-readable storage medium. A computer program is stored on the computer-readable storage medium. When the computer program is run by a processor, the steps of the above DNN model training method are executed.
[0095] Compared with the prior art, the beneficial effect of the computer-readable storage medium provided by this embodiment is the same as the beneficial effect of the DNN model training method provided by the above technical solution, and will not be repeated here.
[0096] Those of ordinary skill in the art can understand that all or part of the steps in the above inventive method can be completed by instructing related hardware through a program. The above program can be stored in a computer-readable storage medium. When the program is executed, it includes: For each step of the method in the above embodiments, the storage medium may be: ROM / RAM, magnetic disk, optical disk, memory card, and the like.
PUM
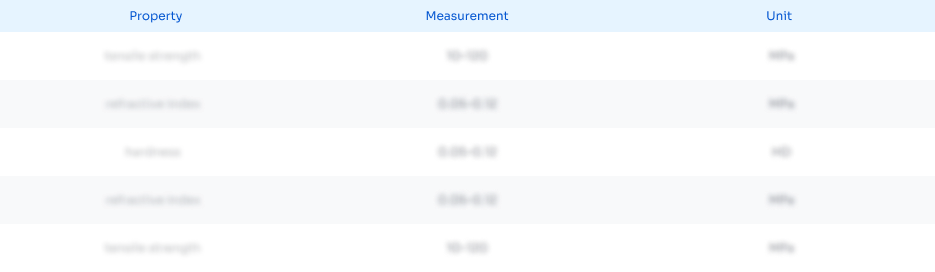
Abstract
Description
Claims
Application Information
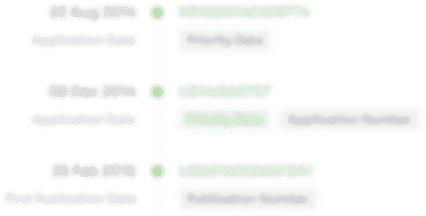
- R&D
- Intellectual Property
- Life Sciences
- Materials
- Tech Scout
- Unparalleled Data Quality
- Higher Quality Content
- 60% Fewer Hallucinations
Browse by: Latest US Patents, China's latest patents, Technical Efficacy Thesaurus, Application Domain, Technology Topic, Popular Technical Reports.
© 2025 PatSnap. All rights reserved.Legal|Privacy policy|Modern Slavery Act Transparency Statement|Sitemap|About US| Contact US: help@patsnap.com