Shape adaptive convolution deep neural network method for hyperspectral image classification
A deep neural network and hyperspectral image technology, applied in the field of shape-adaptive convolutional deep neural network, can solve the problems of loss of detailed information in classification map and poor classification effect, and achieve the effect of excellent generalization and classification performance
- Summary
- Abstract
- Description
- Claims
- Application Information
AI Technical Summary
Problems solved by technology
Method used
Image
Examples
Embodiment
[0053] Hyperspectral images are typical three-dimensional space-spectral data. The simulation experiment uses a set of synthetic hyperspectral data (synthesis dataset) and a set of real hyperspectral data (Indian Pines). The synthetic data set contains 162 spectral bands, the wavelength range is 0.4-2.5 μm, the image size is 200×200, and it contains 5 different types of ground objects, with a total of 40,000 labeled samples. The Indian Pines dataset is a hyperspectral remote sensing image collected by the Airborne Visible Infrared Imaging Spectrometer (AVIRIS) in the Indian Pines Experimental Area, Indiana, USA. The image contains 220 bands in total, the spatial resolution is 20m, and the image size is 145×145. After removing 20 water vapor absorption and low signal-to-noise ratio bands (the band numbers are 104-108, 150-163, 220), the remaining 200 bands were selected as the research objects. This area contains a total of 10366 samples of 16 known features. For the syntheti...
PUM
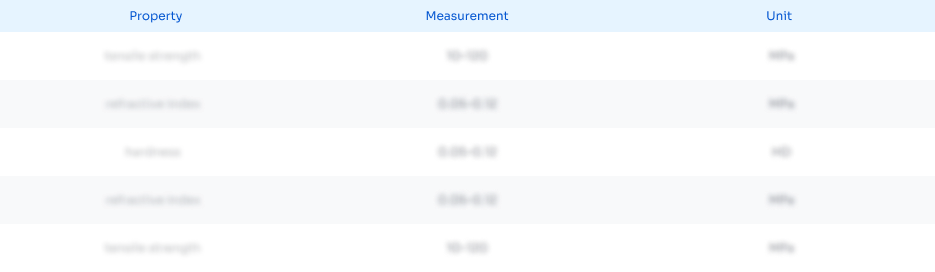
Abstract
Description
Claims
Application Information
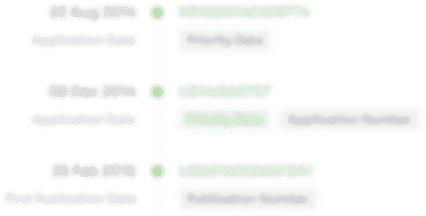
- R&D Engineer
- R&D Manager
- IP Professional
- Industry Leading Data Capabilities
- Powerful AI technology
- Patent DNA Extraction
Browse by: Latest US Patents, China's latest patents, Technical Efficacy Thesaurus, Application Domain, Technology Topic, Popular Technical Reports.
© 2024 PatSnap. All rights reserved.Legal|Privacy policy|Modern Slavery Act Transparency Statement|Sitemap|About US| Contact US: help@patsnap.com