Electrical load identification method based on improved graph convolutional neural network
A convolutional neural network and electricity load technology, applied in neural learning methods, biological neural network models, neural architectures, etc., can solve the problems of poor quality of clustering results, low identification accuracy, and improved intelligence, and improve accuracy. stability and reliability, high identification accuracy, and the effect of avoiding misclassification
- Summary
- Abstract
- Description
- Claims
- Application Information
AI Technical Summary
Problems solved by technology
Method used
Image
Examples
Embodiment Construction
[0045] In order to make the object, technical solution and advantages of the present invention clearer, the present invention will be further described in detail below in conjunction with the accompanying drawings and examples, but the given examples are not intended to limit the present invention.
[0046] As shown in the accompanying drawing (1), the embodiment of the present application provides a graph convolutional neural network user load identification method based on the combination of Euclidean distance and DTW distance. This method specifically includes three sub-processes: The user's electricity consumption data is clustered through a clustering method based on Euclidean distance and DTW distance weighted fusion, and finally identified through a convolutional neural network.
[0047] Specific steps are as follows:
[0048] Step S1: Data collection, collect the electricity load data of the user's electricity consumption place, and make the corresponding electric applia...
PUM
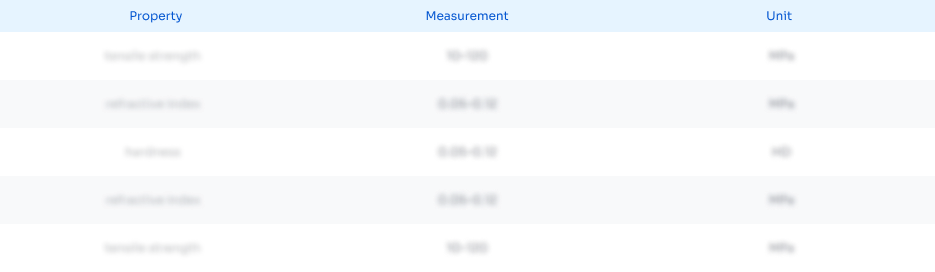
Abstract
Description
Claims
Application Information
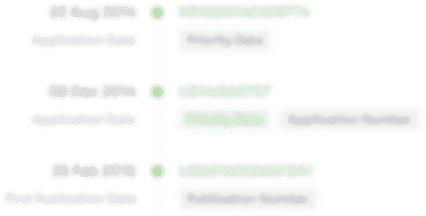
- R&D Engineer
- R&D Manager
- IP Professional
- Industry Leading Data Capabilities
- Powerful AI technology
- Patent DNA Extraction
Browse by: Latest US Patents, China's latest patents, Technical Efficacy Thesaurus, Application Domain, Technology Topic, Popular Technical Reports.
© 2024 PatSnap. All rights reserved.Legal|Privacy policy|Modern Slavery Act Transparency Statement|Sitemap|About US| Contact US: help@patsnap.com