Pedestrian volume prediction system capable of simultaneously modeling space-time dependence and daily flow correlation
A dependency and correlation technology, applied in forecasting, biological neural network models, data processing applications, etc., can solve problems such as ignoring correlations and not being able to capture time-domain change information, and achieve high prediction accuracy
- Summary
- Abstract
- Description
- Claims
- Application Information
AI Technical Summary
Problems solved by technology
Method used
Image
Examples
Embodiment Construction
[0020] Such as figure 2 As shown, this implementation example proposes a prediction system with dual encoder modules based on the codec framework for the multi-step prediction of the city-wide human flow, including:
[0021] ① ST encoder module: It includes two layers of convolutional neural network to capture the spatial dependence of different ranges hierarchically, and a layer of convolutional long-term short-term memory network to capture the dependence of time domain and air domain at the same time. Quantity sequences are encoded as fixed-dimensional representation vectors;
[0022] ②FR encoder module: Capture the correlation of daily flow changes between regions. Firstly, by calculating the similarity of flow changes between regions, the daily flow correlation between regions is obtained, and the correlation of flow changes between regions is generated. is a complete graph of edges, and then uses a graph embedding method to generate a unique representation vector for e...
PUM
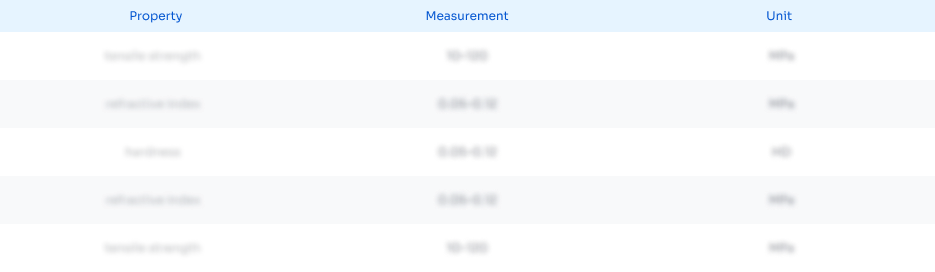
Abstract
Description
Claims
Application Information
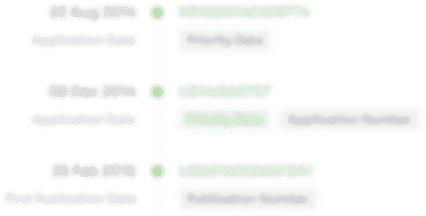
- R&D Engineer
- R&D Manager
- IP Professional
- Industry Leading Data Capabilities
- Powerful AI technology
- Patent DNA Extraction
Browse by: Latest US Patents, China's latest patents, Technical Efficacy Thesaurus, Application Domain, Technology Topic, Popular Technical Reports.
© 2024 PatSnap. All rights reserved.Legal|Privacy policy|Modern Slavery Act Transparency Statement|Sitemap|About US| Contact US: help@patsnap.com