CT image liver tumor segmentation method based on deep learning
A CT image, liver tumor technology, applied in the field of medical image processing, can solve the problem of no fully connected layer, and achieve the effect of improving efficiency and accuracy
- Summary
- Abstract
- Description
- Claims
- Application Information
AI Technical Summary
Problems solved by technology
Method used
Image
Examples
Example Embodiment
[0016] Specific implementation method
[0017] The present invention will be further explained below in conjunction with examples
[0018] The present invention provides a CT image liver tumor segmentation method based on deep learning, using a data set from the LiTS (Liver Tumor Segmentation Challenge, CT image segmentation challenge of liver tumor lesions) data set, and LiTS is a data set for liver tumor segmentation. It contains 131 sets of training data and 70 sets of test data. The training data contains 131 sets of 3D CT images and corresponding 131 sets of real segmentation masks.
[0019] Before using the data, it is necessary to preprocess the CT image data, first convert the CT value of the CT image to the HU value. The scope of the data is limited. In this experiment, the HU value of the atlas is set to include but not limited to [-200, 250], and some irrelevant information and noise are removed. Divide the ROI for cropping, divide the area of the liver, and perform c...
PUM
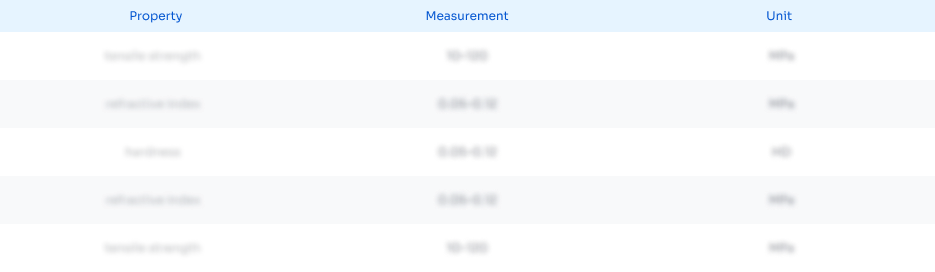
Abstract
Description
Claims
Application Information
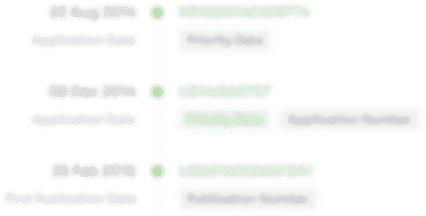
- R&D Engineer
- R&D Manager
- IP Professional
- Industry Leading Data Capabilities
- Powerful AI technology
- Patent DNA Extraction
Browse by: Latest US Patents, China's latest patents, Technical Efficacy Thesaurus, Application Domain, Technology Topic.
© 2024 PatSnap. All rights reserved.Legal|Privacy policy|Modern Slavery Act Transparency Statement|Sitemap