An Image Retrieval System and Method Based on Robust Deep Hash Network
An image retrieval and network technology, which is applied in still image data retrieval, still image data indexing, digital data information retrieval, etc., can solve the problems of poor network robustness and generalization, influence of retrieval results, non-conductivity of discrete space, etc. To achieve the effect of ensuring feature distinction, improving generalization ability, increasing generalization and robustness
- Summary
- Abstract
- Description
- Claims
- Application Information
AI Technical Summary
Problems solved by technology
Method used
Image
Examples
Embodiment
[0108] In order to prove that the robust image hash retrieval method based on the mutual learning mechanism has advantages in performance, the present invention conducts verification and analysis through the following experiments:
[0109] A. Experimental data set
[0110] (1) CIFAR-10
[0111] The CIFAR-10 dataset contains 60,000 32*32 color images, each of which contains 10 categories: airplanes, cars, birds, cats, deer, etc. Each category has 6000 pictures, of which 50000 pictures are the training set and the other 10000 pictures are the test set. The pictures in the CIFAR-10 dataset are single-label data, which means that each picture has one and only one category.
[0112] (2)NUS-WIDE
[0113] The NUS-WIDE dataset contains 269,648 image data collected from Flickr, with a total of 81 categories. Unlike CIFAR-10, the data in NUS-WIDE is multi-label data, that is, each picture may have one or more labels. The present invention only selects the 21 categories with the hig...
PUM
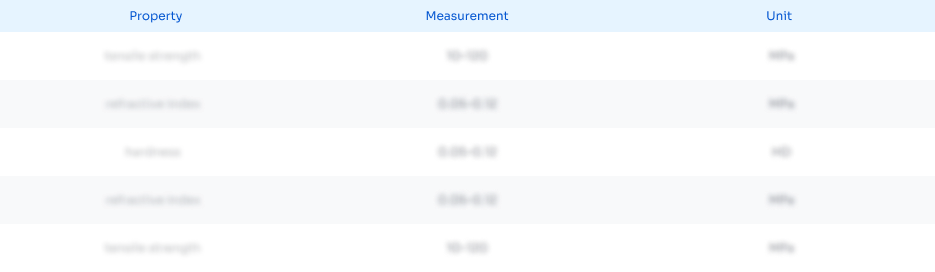
Abstract
Description
Claims
Application Information
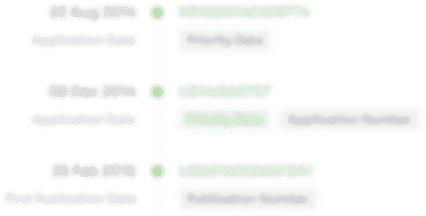
- R&D Engineer
- R&D Manager
- IP Professional
- Industry Leading Data Capabilities
- Powerful AI technology
- Patent DNA Extraction
Browse by: Latest US Patents, China's latest patents, Technical Efficacy Thesaurus, Application Domain, Technology Topic, Popular Technical Reports.
© 2024 PatSnap. All rights reserved.Legal|Privacy policy|Modern Slavery Act Transparency Statement|Sitemap|About US| Contact US: help@patsnap.com