Large-scale multi-view data self-dimension-reduction K-means algorithm and system
A k-means algorithm and multi-view technology, applied in the field of information processing, can solve problems such as ignoring view information complementarity
- Summary
- Abstract
- Description
- Claims
- Application Information
AI Technical Summary
Problems solved by technology
Method used
Image
Examples
Embodiment Construction
[0080] In order to further understand the invention content, characteristics and effects of the present invention, the following examples are given, and detailed descriptions are as follows in conjunction with the accompanying drawings:
[0081] see figure 1 ,
[0082] First preferred embodiment:
[0083] A self-dimension reduction K-means algorithm for large-scale multi-view data, which aims to improve the clustering performance of the traditional multi-view K-means algorithm in high-dimensional data. Fully consider the relationship between features and clustering targets, use the information complementarity between different views, realize the self-reduction of high-dimensional data by finding the optimal subspace on a single view, and use non-negative matrix factorization (NMF) to reduce the loss The function is reconstructed, so that different views share the same clustering indicator matrix, so as to realize the complementarity of multi-view information and complete the...
PUM
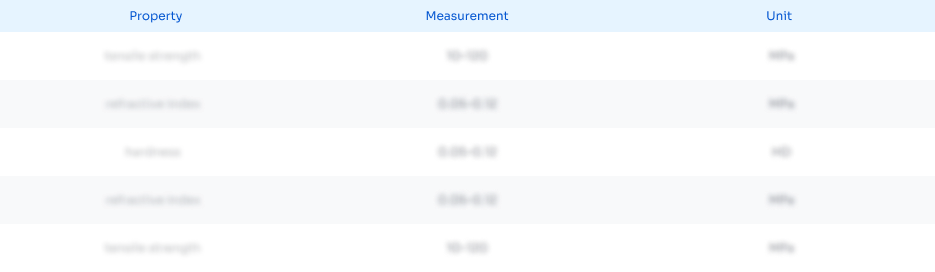
Abstract
Description
Claims
Application Information
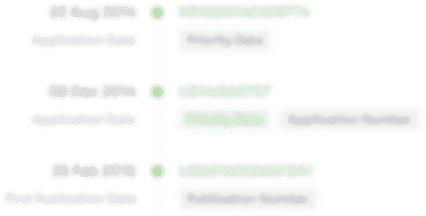
- R&D Engineer
- R&D Manager
- IP Professional
- Industry Leading Data Capabilities
- Powerful AI technology
- Patent DNA Extraction
Browse by: Latest US Patents, China's latest patents, Technical Efficacy Thesaurus, Application Domain, Technology Topic, Popular Technical Reports.
© 2024 PatSnap. All rights reserved.Legal|Privacy policy|Modern Slavery Act Transparency Statement|Sitemap|About US| Contact US: help@patsnap.com