Radar interference signal identification method based on deep CNN integration
A radar jamming and neural network technology, applied in character and pattern recognition, instruments, radio wave measurement systems, etc., can solve overfitting, poor model generalization ability, weak robustness, low recognition accuracy of recognition system, etc. problems, to achieve the effect of improving recognition accuracy and robustness
- Summary
- Abstract
- Description
- Claims
- Application Information
AI Technical Summary
Problems solved by technology
Method used
Image
Examples
specific Embodiment approach 1
[0021] Specific implementation mode one: combine figure 1 To illustrate this embodiment, the radar interference signal recognition method based on deep convolutional neural network integration includes the following steps:
[0022] Step 1. Divide the radar jamming signal time domain data set into three parts: training set, verification set and test set;
[0023] The training set is denoted as X, and the number of training samples is denoted as m;
[0024] The process of dividing the radar interference signal time domain data set in step 1 is as follows:
[0025] Step 11. Mark the original radar data to form a radar interference signal time domain data set: store each sample in a vector, the first 50% of the vector is marked as the real part data of the sample, and the last 50% is marked as the imaginary part of the sample part data;
[0026] Step 12: Randomly divide the radar interference signal time-domain data set into three mutually disjoint sets, which are training set,...
specific Embodiment approach 2
[0037] Embodiment 2: This embodiment provides another solution: CNN is selected as the feature extractor of the radar interference time domain signal, and different classifiers are selected to construct heterogeneous integration. The radar interference signal recognition method based on deep convolutional neural network integration includes the following contents:
[0038] 1. The radar jamming signal time domain data set is divided into three parts: training set, verification set and test set.
[0039] The radar interference signal time-domain data sets used in the present invention include 12 types in total, and the data dimension of each sample is composed of real part data and imaginary part data. In terms of data storage, each sample is stored in a vector, the first 50% of the vector is the real part data of the sample, and the last 50% is the imaginary part data of the sample. For the marked original radar jamming signal time-domain data set, it needs to be randomly divi...
PUM
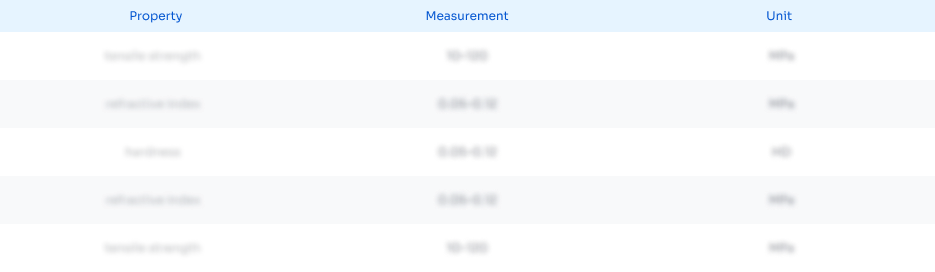
Abstract
Description
Claims
Application Information
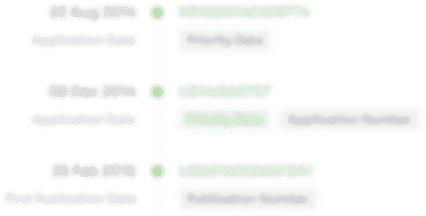
- R&D
- Intellectual Property
- Life Sciences
- Materials
- Tech Scout
- Unparalleled Data Quality
- Higher Quality Content
- 60% Fewer Hallucinations
Browse by: Latest US Patents, China's latest patents, Technical Efficacy Thesaurus, Application Domain, Technology Topic, Popular Technical Reports.
© 2025 PatSnap. All rights reserved.Legal|Privacy policy|Modern Slavery Act Transparency Statement|Sitemap|About US| Contact US: help@patsnap.com