Construction method of boundary forest model, multi-working-condition soft computing model updating method for complex industrial process and application thereof
A technology of forest model and construction method, applied in CAD numerical modeling, design optimization/simulation, etc., can solve problems such as high noise and unreliable predicted values
- Summary
- Abstract
- Description
- Claims
- Application Information
AI Technical Summary
Problems solved by technology
Method used
Image
Examples
Embodiment Construction
[0023]Ensemble learning and online learning methods are one of the latest research directions in the field of machine learning and data mining, and they provide a new measurement mechanism for the field of soft sensing. Aiming at the problems that the leaf nodes of the tree integration model are easy to produce blank areas in the output range, resulting in unreliable predicted values, and the time validity of the data, the invention proposes a related scheme of the key variable online soft sensor technology based on the boundary forest. Among them, by setting the minimum number of samples of different leaf nodes, K tree ensemble models with different leaf node boundaries are formed, and then these trees are fused to cover the blank area of a single tree on the output boundary, which improves the reliability and reliability of the predicted value. accuracy. In addition, facing the characteristics of multi-working conditions in complex industrial processes, a variable-width dy...
PUM
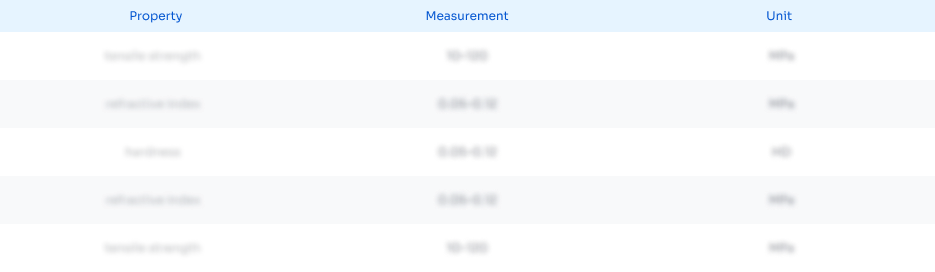
Abstract
Description
Claims
Application Information
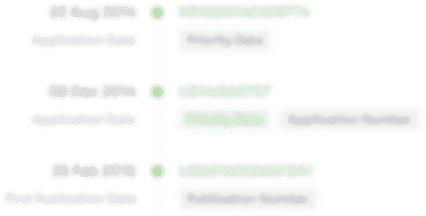
- R&D
- Intellectual Property
- Life Sciences
- Materials
- Tech Scout
- Unparalleled Data Quality
- Higher Quality Content
- 60% Fewer Hallucinations
Browse by: Latest US Patents, China's latest patents, Technical Efficacy Thesaurus, Application Domain, Technology Topic, Popular Technical Reports.
© 2025 PatSnap. All rights reserved.Legal|Privacy policy|Modern Slavery Act Transparency Statement|Sitemap|About US| Contact US: help@patsnap.com