Method for predicting transient stability of power system based on LSTM double-structure model
A technology of power system and prediction method, applied in the direction of electrical components, circuit devices, AC network circuits, etc.
- Summary
- Abstract
- Description
- Claims
- Application Information
AI Technical Summary
Problems solved by technology
Method used
Image
Examples
Embodiment
[0065] figure 1 It is a flow chart of the power system transient stability prediction method based on the LSTM dual structure model of the present invention.
[0066] In this example, if figure 1 As shown, a kind of power system transient stability prediction method based on LSTM dual structure model of the present invention comprises the following steps:
[0067] S1. Use the open source framework Keras to build an LSTM dual-structure model
[0068] Based on the long-short-term memory network LSTM, a variant of the recurrent neural network RNN, and based on the fault characteristics of power system transient faults, a bidirectional long-short-term memory network Bi-Lstm is designed as an LSTM dual-structure model. Among them, the LSTM in Bi-Lstm The number of units is set to n;
[0069] In this embodiment, the bidirectional long-short-term memory network Bi-Lstm is developed from bidirectional RNN (Bi-RNN), solving the problem that RNN can only obtain the previous informati...
example
[0128] Use the power system simulation software to simulate the IEEE39 node system, such as Figure 4 As shown, the IEEE-39 node system includes a total of 10 generators and 39 nodes; under the standard load level, set faults at 20%, 40%, 60%, and 80% of the 34 lines, and the fault types include single-phase Short circuit, two phase short circuit, two phase short circuit to ground, three phase short circuit, three phase short circuit to ground, single phase open circuit. The fault removal time is 0.1s and 0.8s after the fault occurs. Therefore, the number of samples is 34 (number of faulty lines) × 4 (number of positions of each line) × 6 (type of fault) × 2 (time of fault removal) = 1632, a total of 1632 groups of samples were collected, and the data sampling period T = 0.01s. The collected data bus voltage, current, etc., judge the transient stability of the system based on whether the relative power angle difference between any two generators is greater than 360 degrees. ...
PUM
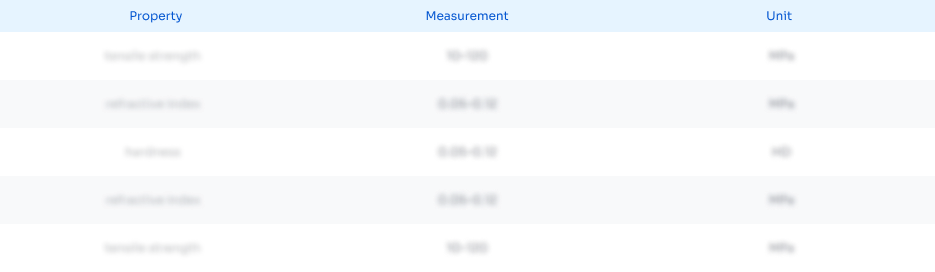
Abstract
Description
Claims
Application Information
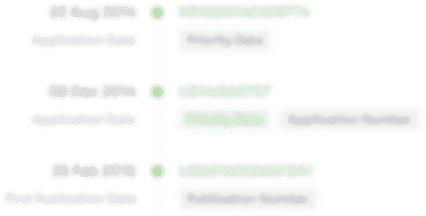
- R&D
- Intellectual Property
- Life Sciences
- Materials
- Tech Scout
- Unparalleled Data Quality
- Higher Quality Content
- 60% Fewer Hallucinations
Browse by: Latest US Patents, China's latest patents, Technical Efficacy Thesaurus, Application Domain, Technology Topic, Popular Technical Reports.
© 2025 PatSnap. All rights reserved.Legal|Privacy policy|Modern Slavery Act Transparency Statement|Sitemap|About US| Contact US: help@patsnap.com