Combined learning method and device based on gradient momentum acceleration
A learning method and momentum technology, applied in the field of joint learning, which can solve problems such as improvement without considering algorithm convergence, slow algorithm convergence, etc.
- Summary
- Abstract
- Description
- Claims
- Application Information
AI Technical Summary
Problems solved by technology
Method used
Image
Examples
Embodiment 1
[0049] like figure 1 Shown is a structural diagram of a joint learning method based on gradient momentum acceleration provided by the present invention. In the figure, the local learning model refers to the machine learning model embedded on each edge node, and the global learning model refers to the global momentum parameter The solution formula of d(t) and the global model parameter w(t), a joint learning method based on gradient momentum acceleration, the joint learning method adopts a distributed system and is applied to image recognition and speech recognition, and the distributed system Including several edge nodes and a central server connecting all edge nodes; the joint learning method includes:
[0050] Step 1: Embed the same machine learning model on each edge node, and execute the momentum gradient descent algorithm in the current aggregation interval to obtain the model parameters and momentum parameters at each moment in the current aggregation interval; the speci...
Embodiment 2
[0074] Corresponding to Embodiment 1 of the present invention, Embodiment 2 of the present invention provides a joint learning device based on gradient momentum acceleration. The joint learning device adopts a distributed system and is applied to image recognition and speech recognition. The distributed system Including several edge nodes and a central server connecting all edge nodes; the united learning device includes:
[0075] The parameter acquisition module is used to divide the training process into several aggregation intervals, each aggregation interval corresponds to the set duration; embed the same machine learning model on each edge node, and execute the momentum gradient descent algorithm in the current aggregation interval Obtain the model parameters and momentum parameters at each moment in the current aggregation interval;
[0076] The aggregation module is used for each edge node to simultaneously send the model parameters and momentum parameters to the centra...
PUM
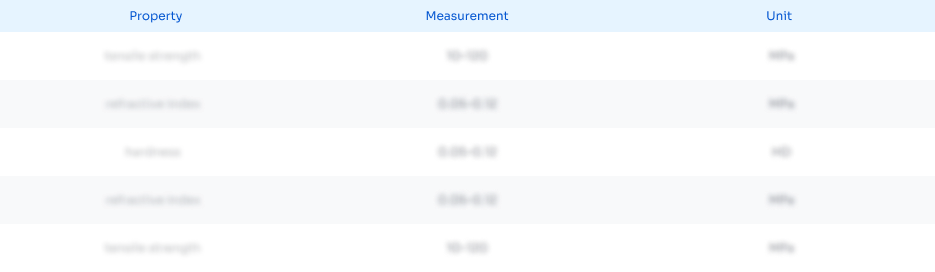
Abstract
Description
Claims
Application Information
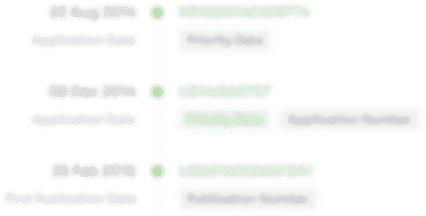
- R&D
- Intellectual Property
- Life Sciences
- Materials
- Tech Scout
- Unparalleled Data Quality
- Higher Quality Content
- 60% Fewer Hallucinations
Browse by: Latest US Patents, China's latest patents, Technical Efficacy Thesaurus, Application Domain, Technology Topic, Popular Technical Reports.
© 2025 PatSnap. All rights reserved.Legal|Privacy policy|Modern Slavery Act Transparency Statement|Sitemap|About US| Contact US: help@patsnap.com