Bearing fault diagnosis method based on hierarchical extreme learning machine
An extreme learning machine and fault diagnosis technology, which is applied in the testing of mechanical components, testing of machine/structural components, measuring devices, etc., can solve problems such as unstable learning effects, and achieve improved recognition accuracy and feature information utilization rate, high The effect of recognition accuracy and fast training speed
- Summary
- Abstract
- Description
- Claims
- Application Information
AI Technical Summary
Problems solved by technology
Method used
Image
Examples
Embodiment 1
[0041] Such as figure 1 As shown, the embodiment of the present invention provides a bearing fault diagnosis method based on hierarchical extreme learning machine. First, the vibration signal of the bearing is decomposed into multiple modes through VMD to achieve the purpose of noise reduction and feature extraction; The SVD algorithm performs secondary feature extraction and data compression on the data of noise reduction and feature extraction; finally, the purpose of fault diagnosis is achieved by learning through the new layered extreme learning machine technology. Specifically include the following steps:
[0042] Step 1. Firstly, the vibration signal data is decomposed by using the VMD (Variational Mode Decomposition) algorithm, and decomposed into K modes. The number of sampling points for each mode is consistent with the original vibration signal. After VMD decomposition, the irregular noise is removed and converged around their respective center frequencies, which ha...
Embodiment 2
[0070] Embodiment 2 of the present invention provides a method of combining VMD-SVD for feature extraction, and finally using a layered extreme learning machine to implement a rolling bearing fault diagnosis method.
[0071] Specific steps are as follows:
[0072] Step 1. The bearing vibration signal is decomposed into multiple modes converging on the center frequency through VMD.
[0073] Step 2: Select the first four items of the decomposed mode and perform SVD decomposition respectively.
[0074] Step 3: Input the features extracted twice into the layered extreme learning machine for training to realize fault diagnosis.
[0075] Hierarchical extreme learning machine is a multi-layer nested improved algorithm of extreme learning machine. In the extreme learning machine algorithm, after specifying the input and output data and the classification type of data (zero regression or complex classification), it can adjust the neural network by itself. weights and thresholds, so t...
PUM
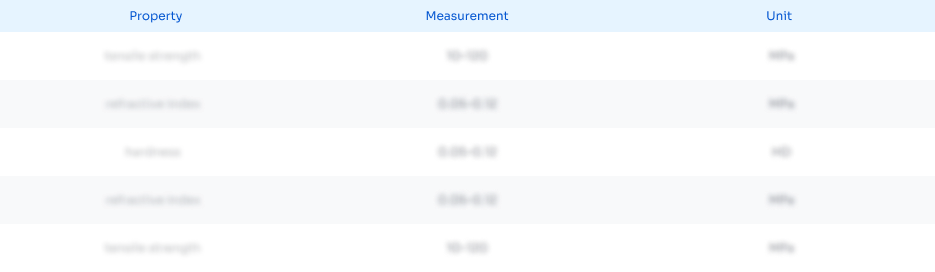
Abstract
Description
Claims
Application Information
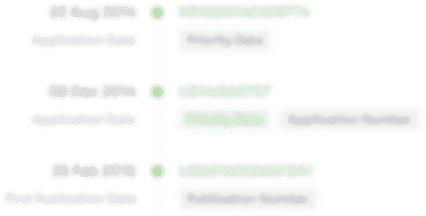
- R&D
- Intellectual Property
- Life Sciences
- Materials
- Tech Scout
- Unparalleled Data Quality
- Higher Quality Content
- 60% Fewer Hallucinations
Browse by: Latest US Patents, China's latest patents, Technical Efficacy Thesaurus, Application Domain, Technology Topic, Popular Technical Reports.
© 2025 PatSnap. All rights reserved.Legal|Privacy policy|Modern Slavery Act Transparency Statement|Sitemap|About US| Contact US: help@patsnap.com