Image defocusing blurring method based on deep learning
A technology of deep learning and fuzzy methods, applied in image enhancement, image analysis, image data processing, etc., can solve problems such as image boundaries and unclear outlines, and achieve clear texture and accurate images
- Summary
- Abstract
- Description
- Claims
- Application Information
AI Technical Summary
Problems solved by technology
Method used
Image
Examples
Embodiment Construction
[0065] For an out-of-focus blurred image taken in a real scene, it is necessary to restore the blurred objects outside the focal plane to obtain a clear image, which can be used figure 2 The defocused deep neural network shown performs image defocused blur processing. Specific steps are as follows.
[0066] (1) Establish a defocus blur dataset
[0067] Construct an out-of-focus blur dataset by shooting or adding random blur, so that each set of data contains a clear image as the original image, and several blurred images as the blurred image corresponding to the clear image.
[0068] (2) Training to remove out-of-focus blur deep neural network
[0069] Randomly select a blurred image I from the deblurred dataset in step (1) blur , and randomly cut out a 256×256 area and input it into the neural network to obtain a 3×256×256 deblurred image P deblur . will deblur the image P deblur and the corresponding sharp image area P in the deblurred data set clear Calculate the no...
PUM
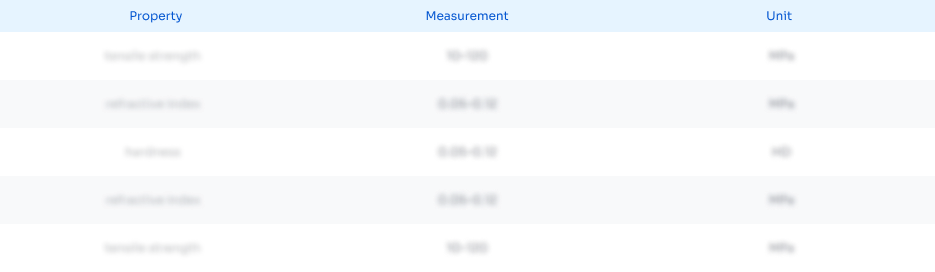
Abstract
Description
Claims
Application Information
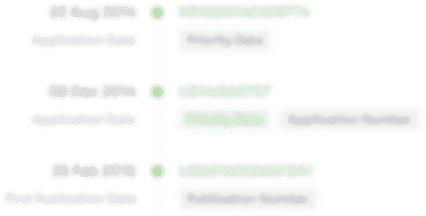
- R&D Engineer
- R&D Manager
- IP Professional
- Industry Leading Data Capabilities
- Powerful AI technology
- Patent DNA Extraction
Browse by: Latest US Patents, China's latest patents, Technical Efficacy Thesaurus, Application Domain, Technology Topic.
© 2024 PatSnap. All rights reserved.Legal|Privacy policy|Modern Slavery Act Transparency Statement|Sitemap